6/4/2024 |
CBT-LLM: A Chinese Large Language Model for Cognitive Behavioral Therapy-based Mental Health Question Answering |
The recent advancements in artificial intelligence highlight the potential of language models in psychological health support. While models trained on data from mental health service platform have achieved preliminary success, challenges persist in areas such as data scarcity, quality, and ensuring a solid foundation in psychological techniques. To address these challenges, this study introduces a novel approach to enhance the precision and efficacy of psychological support through large language models. Specifically, we design a specific prompt derived from principles of Cognitive Behavioral Therapy (CBT) and have generated the CBT QA dataset, specifically for Chinese psychological health Q&A based on CBT structured intervention strategies. Unlike previous methods, our dataset emphasizes professional and structured response. Utilizing this dataset, we fine-tuned the large language model, giving birth to CBT-LLM, the large-scale language model specifically designed for Cognitive Behavioral Therapy techniques. Empirical evaluations demonstrate that CBT-LLM excels in generating structured, professional, and highly relevant responses in psychological health support tasks, showcasing its practicality and quality. The model is available on Hugging Face: this https URL.
|
Hongbin Na |
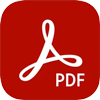
|
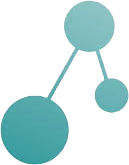 |
|
6/4/2024 |
FREB-TQA: A Fine-Grained Robustness Evaluation Benchmark for Table Question Answering |
Table Question Answering (TQA) aims at composing an answer to a question based on tabular data. While prior research has shown that TQA models lack robustness, understanding the underlying cause and nature of this issue remains predominantly unclear, posing a significant obstacle to the development of robust TQA systems. In this paper, we formalize three major desiderata for a fine-grained evaluation of robustness of TQA systems. They should (i) answer questions regardless of alterations in table structure, (ii) base their responses on the content of relevant cells rather than on biases, and (iii) demonstrate robust numerical reasoning capabilities. To investigate these aspects, we create and publish a novel TQA evaluation benchmark in English. Our extensive experimental analysis reveals that none of the examined state-of-the-art TQA systems consistently excels in these three aspects. Our benchmark is a crucial instrument for monitoring the behavior of TQA systems and paves the way for the development of robust TQA systems. We release our benchmark publicly.
|
Wei Zhou, Mohsen Mesgar, Heike Adel, Annemarie Friedrich |
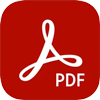
|
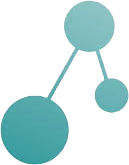 |
|
6/4/2024 |
LISA: Layerwise Importance Sampling for Memory-Efficient Large Language Model Fine-Tuning |
The machine learning community has witnessed impressive advancements since the first appearance of large language models (LLMs), yet their huge memory consumption has become a major roadblock to large-scale training. Parameter Efficient Fine-Tuning techniques such as Low-Rank Adaptation (LoRA) have been proposed to alleviate this problem, but their performance still fails to match full parameter training in most large-scale fine-tuning settings. Attempting to complement this deficiency, we investigate layerwise properties of LoRA on fine-tuning tasks and observe an uncommon skewness of weight norms across different layers. Utilizing this key observation, a surprisingly simple training strategy is discovered, which outperforms both LoRA and full parameter training in a wide range of settings with memory costs as low as LoRA. We name it Layerwise Importance Sampled AdamW (LISA), a promising alternative for LoRA, which applies the idea of importance sampling to different layers in LLMs and randomly freeze most middle layers during optimization. Experimental results show that with similar or less GPU memory consumption, LISA surpasses LoRA or even full parameter tuning in downstream fine-tuning tasks, where LISA consistently outperforms LoRA by over $11\%$-$37\%$ in terms of MT-Bench scores. On large models, specifically LLaMA-2-70B, LISA achieves on-par or better performance than LoRA on MT-Bench, GSM8K, and PubMedQA, demonstrating its effectiveness across different domains.
|
Rui Pan, Xiang Liu, Shizhe Diao, Renjie Pi, Jipeng Zhang, Chi Han, Tong Zhang |
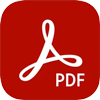
|
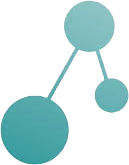 |
|
6/4/2024 |
VoiceCraft: Zero-Shot Speech Editing and Text-to-Speech in the Wild |
We introduce VoiceCraft, a token infilling neural codec language model, that achieves state-of-the-art performance on both speech editing and zero-shot text-to-speech (TTS) on audiobooks, internet videos, and podcasts. VoiceCraft employs a Transformer decoder architecture and introduces a token rearrangement procedure that combines causal masking and delayed stacking to enable generation within an existing sequence. On speech editing tasks, VoiceCraft produces edited speech that is nearly indistinguishable from unedited recordings in terms of naturalness, as evaluated by humans; for zero-shot TTS, our model outperforms prior SotA models including VALLE and the popular commercial model XTTS-v2. Crucially, the models are evaluated on challenging and realistic datasets, that consist of diverse accents, speaking styles, recording conditions, and background noise and music, and our model performs consistently well compared to other models and real recordings. In particular, for speech editing evaluation, we introduce a high quality, challenging, and realistic dataset named RealEdit. We encourage readers to listen to the demos at this https URL.
|
Puyuan Peng, Po-Yao Huang, Daniel Li, Abdelrahman Mohamed, David Harwath |
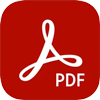
|
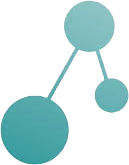 |
|
6/4/2024 |
CatLIP: CLIP-level Visual Recognition Accuracy with 2.7x Faster Pre-training on Web-scale Image-Text Data |
Contrastive learning has emerged as a transformative method for learning effective visual representations through the alignment of image and text embeddings. However, pairwise similarity computation in contrastive loss between image and text pairs poses computational challenges. This paper presents a novel weakly supervised pre-training of vision models on web-scale image-text data. The proposed method reframes pre-training on image-text data as a classification task. Consequently, it eliminates the need for pairwise similarity computations in contrastive loss, achieving a remarkable $2.7\times$ acceleration in training speed compared to contrastive learning on web-scale data. Through extensive experiments spanning diverse vision tasks, including detection and segmentation, we demonstrate that the proposed method maintains high representation quality. Our source code along with pre-trained model weights and training recipes is available at \url{this https URL}.
|
Sachin Mehta, Maxwell Horton, Fartash Faghri, Mohammad Hossein Sekhavat, Mahyar Najibi, Mehrdad Farajtabar, Oncel Tuzel, Mohammad Rastegari |
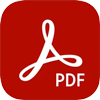
|
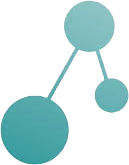 |
|
6/4/2024 |
OpenELM: An Efficient Language Model Family with Open-source Training and Inference Framework |
The reproducibility and transparency of large language models are crucial for advancing open research, ensuring the trustworthiness of results, and enabling investigations into data and model biases, as well as potential risks. To this end, we release OpenELM, a state-of-the-art open language model. OpenELM uses a layer-wise scaling strategy to efficiently allocate parameters within each layer of the transformer model, leading to enhanced accuracy. For example, with a parameter budget of approximately one billion parameters, OpenELM exhibits a 2.36% improvement in accuracy compared to OLMo while requiring $2\times$ fewer pre-training tokens. Diverging from prior practices that only provide model weights and inference code, and pre-train on private datasets, our release includes the complete framework for training and evaluation of the language model on publicly available datasets, including training logs, multiple checkpoints, and pre-training configurations. We also release code to convert models to MLX library for inference and fine-tuning on Apple devices. This comprehensive release aims to empower and strengthen the open research community, paving the way for future open research endeavors. Our source code along with pre-trained model weights and training recipes is available at \url{this https URL}. Additionally, \model models can be found on HuggingFace at: \url{this https URL}.
|
Sachin Mehta, Mohammad Hossein Sekhavat, Qingqing Cao, Maxwell Horton, Yanzi Jin, Chenfan Sun, Iman Mirzadeh, Mahyar Najibi, Dmitry Belenko, Peter Zatloukal, Mohammad Rastegari |
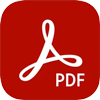
|
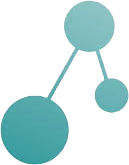 |
|
6/4/2024 |
Extending Llama-3's Context Ten-Fold Overnight |
We extend the context length of Llama-3-8B-Instruct from 8K to 80K via QLoRA fine-tuning. The entire training cycle is super efficient, which takes 8 hours on one 8xA800 (80G) GPU machine. The resulted model exhibits superior performances across a broad range of evaluation tasks, such as NIHS, topic retrieval, and long-context language understanding; meanwhile, it also well preserves the original capability over short contexts. The dramatic context extension is mainly attributed to merely 3.5K synthetic training samples generated by GPT-4 , which indicates the LLMs' inherent (yet largely underestimated) potential to extend its original context length. In fact, the context length could be extended far beyond 80K with more computation resources. Therefore, the team will publicly release the entire resources (including data, model, data generation pipeline, training code) so as to facilitate the future research from the community: \url{this https URL}.
|
Peitian Zhang, Ninglu Shao, Zheng Liu, Shitao Xiao, Hongjin Qian, Qiwei Ye, Zhicheng Dou |
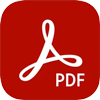
|
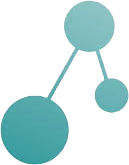 |
|
6/4/2024 |
Computational Job Market Analysis with Natural Language Processing |
[Abridged Abstract] Recent technological advances underscore labor market dynamics, yielding significant consequences for employment prospects and increasing job vacancy data across platforms and languages. Aggregating such data holds potential for valuable insights into labor market demands, new skills emergence, and facilitating job matching for various stakeholders. However, despite prevalent insights in the private sector, transparent language technology systems and data for this domain are lacking. This thesis investigates Natural Language Processing (NLP) technology for extracting relevant information from job descriptions, identifying challenges including scarcity of training data, lack of standardized annotation guidelines, and shortage of effective extraction methods from job ads. We frame the problem, obtaining annotated data, and introducing extraction methodologies. Our contributions include job description datasets, a de-identification dataset, and a novel active learning algorithm for efficient model training. We propose skill extraction using weak supervision, a taxonomy-aware pre-training methodology adapting multilingual language models to the job market domain, and a retrieval-augmented model leveraging multiple skill extraction datasets to enhance overall performance. Finally, we ground extracted information within a designated taxonomy.
|
Mike Zhang |
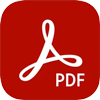
|
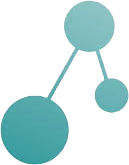 |
|
6/4/2024 |
Mini-Gemini: Mining the Potential of Multi-modality Vision Language Models |
In this work, we introduce Mini-Gemini, a simple and effective framework enhancing multi-modality Vision Language Models (VLMs). Despite the advancements in VLMs facilitating basic visual dialog and reasoning, a performance gap persists compared to advanced models like GPT-4 and Gemini. We try to narrow the gap by mining the potential of VLMs for better performance and any-to-any workflow from three aspects, i.e., high-resolution visual tokens, high-quality data, and VLM-guided generation. To enhance visual tokens, we propose to utilize an additional visual encoder for high-resolution refinement without increasing the visual token count. We further construct a high-quality dataset that promotes precise image comprehension and reasoning-based generation, expanding the operational scope of current VLMs. In general, Mini-Gemini further mines the potential of VLMs and empowers current frameworks with image understanding, reasoning, and generation simultaneously. Mini-Gemini supports a series of dense and MoE Large Language Models (LLMs) from 2B to 34B. It is demonstrated to achieve leading performance in several zero-shot benchmarks and even surpasses the developed private models. Code and models are available at this https URL.
|
Yanwei Li, Yuechen Zhang, Chengyao Wang, Zhisheng Zhong, Yixin Chen, Ruihang Chu, Shaoteng Liu, Jiaya Jia |
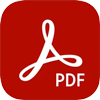
|
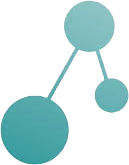 |
|
6/4/2024 |
AIOS: LLM Agent Operating System |
The integration and deployment of large language model (LLM)-based intelligent agents have been fraught with challenges that compromise their efficiency and efficacy. Among these issues are sub-optimal scheduling and resource allocation of agent requests over the LLM, the difficulties in maintaining context during interactions between agent and LLM, and the complexities inherent in integrating heterogeneous agents with different capabilities and specializations. The rapid increase of agent quantity and complexity further exacerbates these issues, often leading to bottlenecks and sub-optimal utilization of resources. Inspired by these challenges, this paper presents AIOS, an LLM agent operating system, which embeds large language model into operating systems (OS) as the brain of the OS, enabling an operating system "with soul" -- an important step towards AGI. Specifically, AIOS is designed to optimize resource allocation, facilitate context switch across agents, enable concurrent execution of agents, provide tool service for agents, and maintain access control for agents. We present the architecture of such an operating system, outline the core challenges it aims to resolve, and provide the basic design and implementation of the AIOS. Our experiments on concurrent execution of multiple agents demonstrate the reliability and efficiency of our AIOS modules. Through this, we aim to not only improve the performance and efficiency of LLM agents but also to pioneer for better development and deployment of the AIOS ecosystem in the future. The project is open-source at this https URL.
|
Kai Mei, Zelong Li, Shuyuan Xu, Ruosong Ye, Yingqiang Ge, Yongfeng Zhang |
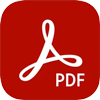
|
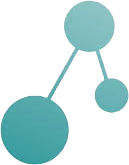 |
|
6/4/2024 |
Better Synthetic Data by Retrieving and Transforming Existing Datasets |
Despite recent advances in large language models, building dependable and deployable NLP models typically requires abundant, high-quality training data. However, task-specific data is not available for many use cases, and manually curating task-specific data is labor-intensive. Recent work has studied prompt-driven synthetic data generation using large language models, but these generated datasets tend to lack complexity and diversity. To address these limitations, we introduce a method, DataTune, to make better use of existing, publicly available datasets to improve automatic dataset generation. DataTune performs dataset transformation, enabling the repurposing of publicly available datasets into a format that is directly aligned with the specific requirements of target tasks. On a diverse set of language-based tasks from the BIG-Bench benchmark, we find that finetuning language models via DataTune improves over a few-shot prompting baseline by 49% and improves over existing methods that use synthetic or retrieved training data by 34%. We find that dataset transformation significantly increases the diversity and difficulty of generated data on many tasks. We integrate DataTune into an open-source repository to make this method accessible to the community: this https URL.
|
Saumya Gandhi, Ritu Gala, Vijay Viswanathan, Tongshuang Wu, Graham Neubig |
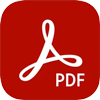
|
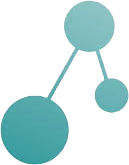 |
|
6/4/2024 |
MoDE: CLIP Data Experts via Clustering |
The success of contrastive language-image pretraining (CLIP) relies on the supervision from the pairing between images and captions, which tends to be noisy in web-crawled data. We present Mixture of Data Experts (MoDE) and learn a system of CLIP data experts via clustering. Each data expert is trained on one data cluster, being less sensitive to false negative noises in other clusters. At inference time, we ensemble their outputs by applying weights determined through the correlation between task metadata and cluster conditions. To estimate the correlation precisely, the samples in one cluster should be semantically similar, but the number of data experts should still be reasonable for training and inference. As such, we consider the ontology in human language and propose to use fine-grained cluster centers to represent each data expert at a coarse-grained level. Experimental studies show that four CLIP data experts on ViT-B/16 outperform the ViT-L/14 by OpenAI CLIP and OpenCLIP on zero-shot image classification but with less ($<$35\%) training cost. Meanwhile, MoDE can train all data expert asynchronously and can flexibly include new data experts. The code is available at this https URL.
|
Jiawei Ma, Po-Yao Huang, Saining Xie, Shang-Wen Li, Luke Zettlemoyer, Shih-Fu Chang, Wen-Tau Yih, Hu Xu |
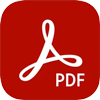
|
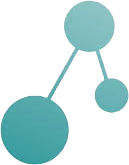 |
|
6/4/2024 |
Fine-Tuning Language Models with Reward Learning on Policy |
Reinforcement learning from human feedback (RLHF) has emerged as an effective approach to aligning large language models (LLMs) to human preferences. RLHF contains three steps, i.e., human preference collecting, reward learning, and policy optimization, which are usually performed serially. Despite its popularity, however, (fixed) reward models may suffer from inaccurate off-distribution, since policy optimization continuously shifts LLMs' data distribution. Repeatedly collecting new preference data from the latest LLMs may alleviate this issue, which unfortunately makes the resulting system more complicated and difficult to optimize. In this paper, we propose reward learning on policy (RLP), an unsupervised framework that refines a reward model using policy samples to keep it on-distribution. Specifically, an unsupervised multi-view learning method is introduced to learn robust representations of policy samples. Meanwhile, a synthetic preference generation approach is developed to simulate high-quality preference data with policy outputs. Extensive experiments on three benchmark datasets show that RLP consistently outperforms the state-of-the-art. Our code is available at \url{this https URL}.
|
Hao Lang, Fei Huang, Yongbin Li |
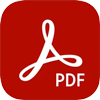
|
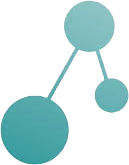 |
|
6/4/2024 |
A Survey on Self-Evolution of Large Language Models |
Large language models (LLMs) have significantly advanced in various fields and intelligent agent applications. However, current LLMs that learn from human or external model supervision are costly and may face performance ceilings as task complexity and diversity increase. To address this issue, self-evolution approaches that enable LLM to autonomously acquire, refine, and learn from experiences generated by the model itself are rapidly growing. This new training paradigm inspired by the human experiential learning process offers the potential to scale LLMs towards superintelligence. In this work, we present a comprehensive survey of self-evolution approaches in LLMs. We first propose a conceptual framework for self-evolution and outline the evolving process as iterative cycles composed of four phases: experience acquisition, experience refinement, updating, and evaluation. Second, we categorize the evolution objectives of LLMs and LLM-based agents; then, we summarize the literature and provide taxonomy and insights for each module. Lastly, we pinpoint existing challenges and propose future directions to improve self-evolution frameworks, equipping researchers with critical insights to fast-track the development of self-evolving LLMs.
|
Zhengwei Tao, Ting-En Lin, Xiancai Chen, Hangyu Li, Yuchuan Wu, Yongbin Li, Zhi Jin, Fei Huang, Dacheng Tao, Jingren Zhou |
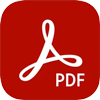
|
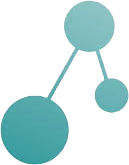 |
|
6/4/2024 |
StreamingT2V: Consistent, Dynamic, and Extendable Long Video Generation from Text |
Text-to-video diffusion models enable the generation of high-quality videos that follow text instructions, making it easy to create diverse and individual content. However, existing approaches mostly focus on high-quality short video generation (typically 16 or 24 frames), ending up with hard-cuts when naively extended to the case of long video synthesis. To overcome these limitations, we introduce StreamingT2V, an autoregressive approach for long video generation of 80, 240, 600, 1200 or more frames with smooth transitions. The key components are:(i) a short-term memory block called conditional attention module (CAM), which conditions the current generation on the features extracted from the previous chunk via an attentional mechanism, leading to consistent chunk transitions, (ii) a long-term memory block called appearance preservation module, which extracts high-level scene and object features from the first video chunk to prevent the model from forgetting the initial scene, and (iii) a randomized blending approach that enables to apply a video enhancer autoregressively for infinitely long videos without inconsistencies between chunks. Experiments show that StreamingT2V generates high motion amount. In contrast, all competing image-to-video methods are prone to video stagnation when applied naively in an autoregressive manner. Thus, we propose with StreamingT2V a high-quality seamless text-to-long video generator that outperforms competitors with consistency and motion. Our code will be available at: this https URL
|
Roberto Henschel, Levon Khachatryan, Daniil Hayrapetyan, Hayk Poghosyan, Vahram Tadevosyan, Zhangyang Wang, Shant Navasardyan, Humphrey Shi |
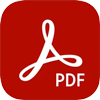
|
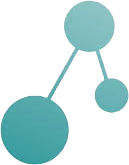 |
|
6/4/2024 |
Xwin-LM: Strong and Scalable Alignment Practice for LLMs |
In this work, we present Xwin-LM, a comprehensive suite of alignment methodologies for large language models (LLMs). This suite encompasses several key techniques, including supervised finetuning (SFT), reward modeling (RM), rejection sampling finetuning (RS), and direct preference optimization (DPO). The key components are as follows: (1) Xwin-LM-SFT, models initially finetuned with high-quality instruction data; (2) Xwin-Pair, a large-scale, multi-turn preference dataset meticulously annotated using GPT-4; (3) Xwin-RM, reward models trained on Xwin-Pair, developed at scales of 7B, 13B, and 70B parameters; (4) Xwin-Set, a multiwise preference dataset in which each prompt is linked to 64 unique responses generated by Xwin-LM-SFT and scored by Xwin-RM; (5) Xwin-LM-RS, models finetuned with the highest-scoring responses from Xwin-Set; (6) Xwin-LM-DPO, models further optimized on Xwin-Set using the DPO algorithm. Our evaluations on AlpacaEval and MT-bench demonstrate consistent and significant improvements across the pipeline, demonstrating the strength and scalability of Xwin-LM. The repository this https URL will be continually updated to foster community research.
|
Bolin Ni, JingCheng Hu, Yixuan Wei, Houwen Peng, Zheng Zhang, Gaofeng Meng, Han Hu |
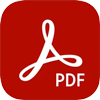
|
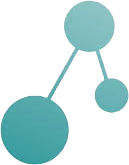 |
|
6/4/2024 |
Tango 2: Aligning Diffusion-based Text-to-Audio Generations through Direct Preference Optimization |
Generative multimodal content is increasingly prevalent in much of the content creation arena, as it has the potential to allow artists and media personnel to create pre-production mockups by quickly bringing their ideas to life. The generation of audio from text prompts is an important aspect of such processes in the music and film industry. Many of the recent diffusion-based text-to-audio models focus on training increasingly sophisticated diffusion models on a large set of datasets of prompt-audio pairs. These models do not explicitly focus on the presence of concepts or events and their temporal ordering in the output audio with respect to the input prompt. Our hypothesis is focusing on how these aspects of audio generation could improve audio generation performance in the presence of limited data. As such, in this work, using an existing text-to-audio model Tango, we synthetically create a preference dataset where each prompt has a winner audio output and some loser audio outputs for the diffusion model to learn from. The loser outputs, in theory, have some concepts from the prompt missing or in an incorrect order. We fine-tune the publicly available Tango text-to-audio model using diffusion-DPO (direct preference optimization) loss on our preference dataset and show that it leads to improved audio output over Tango and AudioLDM2, in terms of both automatic- and manual-evaluation metrics.
|
Navonil Majumder, Chia-Yu Hung, Deepanway Ghosal, Wei-Ning Hsu, Rada Mihalcea, Soujanya Poria |
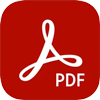
|
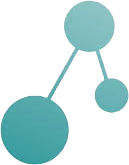 |
|
6/4/2024 |
Sample Design Engineering: An Empirical Study of What Makes Good Downstream Fine-Tuning Samples for LLMs |
In the burgeoning field of Large Language Models (LLMs) like ChatGPT and LLaMA, Prompt Engineering (PE) is renowned for boosting zero-shot or in-context learning (ICL) through prompt modifications. Yet, the realm of the sample design for downstream fine-tuning, crucial for task-specific LLM adaptation, is largely unexplored. This paper introduces Sample Design Engineering (SDE), a methodical approach to enhancing LLMs' post-tuning performance by refining input, output, and reasoning designs. We conduct a series of in-domain (ID) and out-of-domain (OOD) experiments to assess the impact of various design options on LLMs' downstream performance, revealing several intriguing patterns that hold consistently across different LLMs. Based on these insights, we propose an integrated SDE strategy, combining the most effective options, and validate its consistent superiority over heuristic sample designs in complex downstream tasks like multi-aspect sentiment analysis, event extraction, and nested entity recognition. Additionally, analyses of LLMs' inherent prompt/output perplexity, zero-shot, and ICL abilities illustrate that good PE strategies may not always translate to good SDE strategies. Code available at this https URL.
|
Biyang Guo, He Wang, Wenyilin Xiao, Hong Chen, Zhuxin Lee, Songqiao Han, Hailiang Huang |
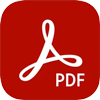
|
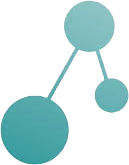 |
|
6/4/2024 |
ReFT: Representation Finetuning for Language Models |
Parameter-efficient fine-tuning (PEFT) methods seek to adapt large models via updates to a small number of weights. However, much prior interpretability work has shown that representations encode rich semantic information, suggesting that editing representations might be a more powerful alternative. Here, we pursue this hypothesis by developing a family of $\textbf{Representation Finetuning (ReFT)}$ methods. ReFT methods operate on a frozen base model and learn task-specific interventions on hidden representations. We define a strong instance of the ReFT family, Low-rank Linear Subspace ReFT (LoReFT). LoReFT is a drop-in replacement for existing PEFTs and learns interventions that are 10x-50x more parameter-efficient than prior state-of-the-art PEFTs. We showcase LoReFT on eight commonsense reasoning tasks, four arithmetic reasoning tasks, Alpaca-Eval v1.0, and GLUE. In all these evaluations, LoReFT delivers the best balance of efficiency and performance, and almost always outperforms state-of-the-art PEFTs. We release a generic ReFT training library publicly at this https URL.
|
Zhengxuan Wu, Aryaman Arora, Zheng Wang, Atticus Geiger, Dan Jurafsky, Christopher D. Manning, Christopher Potts |
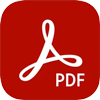
|
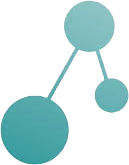 |
|
6/4/2024 |
MING-MOE: Enhancing Medical Multi-Task Learning in Large Language Models with Sparse Mixture of Low-Rank Adapter Experts |
Large language models like ChatGPT have shown substantial progress in natural language understanding and generation, proving valuable across various disciplines, including the medical field. Despite advancements, challenges persist due to the complexity and diversity inherent in medical tasks which often require multi-task learning capabilities. Previous approaches, although beneficial, fall short in real-world applications because they necessitate task-specific annotations at inference time, limiting broader generalization. This paper introduces MING-MOE, a novel Mixture-of-Expert~(MOE)-based medical large language model designed to manage diverse and complex medical tasks without requiring task-specific annotations, thus enhancing its usability across extensive datasets. MING-MOE employs a Mixture of Low-Rank Adaptation (MoLoRA) technique, allowing for efficient parameter usage by maintaining base model parameters static while adapting through a minimal set of trainable parameters. We demonstrate that MING-MOE achieves state-of-the-art (SOTA) performance on over 20 medical tasks, illustrating a significant improvement over existing models. This approach not only extends the capabilities of medical language models but also improves inference efficiency.
|
Yusheng Liao, Shuyang Jiang, Yu Wang, Yanfeng Wang |
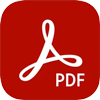
|
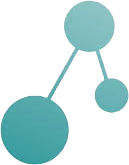 |
|
6/4/2024 |
Prometheus 2: An Open Source Language Model Specialized in Evaluating Other Language Models |
Proprietary LMs such as GPT-4 are often employed to assess the quality of responses from various LMs. However, concerns including transparency, controllability, and affordability strongly motivate the development of open-source LMs specialized in evaluations. On the other hand, existing open evaluator LMs exhibit critical shortcomings: 1) they issue scores that significantly diverge from those assigned by humans, and 2) they lack the flexibility to perform both direct assessment and pairwise ranking, the two most prevalent forms of assessment. Additionally, they do not possess the ability to evaluate based on custom evaluation criteria, focusing instead on general attributes like helpfulness and harmlessness. To address these issues, we introduce Prometheus 2, a more powerful evaluator LM than its predecessor that closely mirrors human and GPT-4 judgements. Moreover, it is capable of processing both direct assessment and pair-wise ranking formats grouped with a user-defined evaluation criteria. On four direct assessment benchmarks and four pairwise ranking benchmarks, Prometheus 2 scores the highest correlation and agreement with humans and proprietary LM judges among all tested open evaluator LMs. Our models, code, and data are all publicly available at this https URL.
|
Seungone Kim, Juyoung Suk, Shayne Longpre, Bill Yuchen Lin, Jamin Shin, Sean Welleck, Graham Neubig, Moontae Lee, Kyungjae Lee, Minjoon Seo |
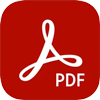
|
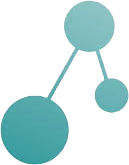 |
|
6/4/2024 |
AutoWebGLM: Bootstrap And Reinforce A Large Language Model-based Web Navigating Agent |
Large language models (LLMs) have fueled many intelligent agent tasks, such as web navigation -- but most existing agents perform far from satisfying in real-world webpages due to three factors: (1) the versatility of actions on webpages, (2) HTML text exceeding model processing capacity, and (3) the complexity of decision-making due to the open-domain nature of web. In light of the challenge, we develop AutoWebGLM, a GPT-4-outperforming automated web navigation agent built upon ChatGLM3-6B. Inspired by human browsing patterns, we design an HTML simplification algorithm to represent webpages, preserving vital information succinctly. We employ a hybrid human-AI method to build web browsing data for curriculum training. Then, we bootstrap the model by reinforcement learning and rejection sampling to further facilitate webpage comprehension, browser operations, and efficient task decomposition by itself. For testing, we establish a bilingual benchmark -- AutoWebBench -- for real-world web browsing tasks. We evaluate AutoWebGLM across diverse web navigation benchmarks, revealing its improvements but also underlying challenges to tackle real environments. Related code, model, and data will be released at \url{this https URL}.
|
Hanyu Lai, Xiao Liu, Iat Long Iong, Shuntian Yao, Yuxuan Chen, Pengbo Shen, Hao Yu, Hanchen Zhang, Xiaohan Zhang, Yuxiao Dong, Jie Tang |
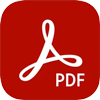
|
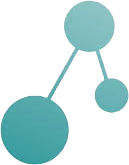 |
|
6/4/2024 |
Long-form factuality in large language models |
Large language models (LLMs) often generate content that contains factual errors when responding to fact-seeking prompts on open-ended topics. To benchmark a model's long-form factuality in open domains, we first use GPT-4 to generate LongFact, a prompt set comprising thousands of questions spanning 38 topics. We then propose that LLM agents can be used as automated evaluators for long-form factuality through a method which we call Search-Augmented Factuality Evaluator (SAFE). SAFE utilizes an LLM to break down a long-form response into a set of individual facts and to evaluate the accuracy of each fact using a multi-step reasoning process comprising sending search queries to Google Search and determining whether a fact is supported by the search results. Furthermore, we propose extending F1 score as an aggregated metric for long-form factuality. To do so, we balance the percentage of supported facts in a response (precision) with the percentage of provided facts relative to a hyperparameter representing a user's preferred response length (recall). Empirically, we demonstrate that LLM agents can achieve superhuman rating performance - on a set of ~16k individual facts, SAFE agrees with crowdsourced human annotators 72% of the time, and on a random subset of 100 disagreement cases, SAFE wins 76% of the time. At the same time, SAFE is more than 20 times cheaper than human annotators. We also benchmark thirteen language models on LongFact across four model families (Gemini, GPT, Claude, and PaLM-2), finding that larger language models generally achieve better long-form factuality. LongFact, SAFE, and all experimental code are available at this https URL.
|
Jerry Wei, Chengrun Yang, Xinying Song, Yifeng Lu, Nathan Hu, Dustin Tran, Daiyi Peng, Ruibo Liu, Da Huang, Cosmo Du, Quoc V. Le |
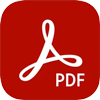
|
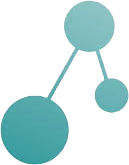 |
|
6/4/2024 |
State Space Model for New-Generation Network Alternative to Transformers: A Survey |
In the post-deep learning era, the Transformer architecture has demonstrated its powerful performance across pre-trained big models and various downstream tasks. However, the enormous computational demands of this architecture have deterred many researchers. To further reduce the complexity of attention models, numerous efforts have been made to design more efficient methods. Among them, the State Space Model (SSM), as a possible replacement for the self-attention based Transformer model, has drawn more and more attention in recent years. In this paper, we give the first comprehensive review of these works and also provide experimental comparisons and analysis to better demonstrate the features and advantages of SSM. Specifically, we first give a detailed description of principles to help the readers quickly capture the key ideas of SSM. After that, we dive into the reviews of existing SSMs and their various applications, including natural language processing, computer vision, graph, multi-modal and multi-media, point cloud/event stream, time series data, and other domains. In addition, we give statistical comparisons and analysis of these models and hope it helps the readers to understand the effectiveness of different structures on various tasks. Then, we propose possible research points in this direction to better promote the development of the theoretical model and application of SSM. More related works will be continuously updated on the following GitHub: this https URL.
|
Xiao Wang, Shiao Wang, Yuhe Ding, Yuehang Li, Wentao Wu, Yao Rong, Weizhe Kong, Ju Huang, Shihao Li, Haoxiang Yang, Ziwen Wang, Bo Jiang, Chenglong Li, Yaowei Wang, Yonghong Tian, Jin Tang |
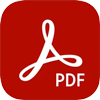
|
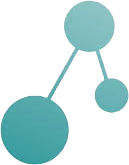 |
|
6/4/2024 |
Dataverse: Open-Source ETL (Extract, Transform, Load) Pipeline for Large Language Models |
To address the challenges associated with data processing at scale, we propose Dataverse, a unified open-source Extract-Transform-Load (ETL) pipeline for large language models (LLMs) with a user-friendly design at its core. Easy addition of custom processors with block-based interface in Dataverse allows users to readily and efficiently use Dataverse to build their own ETL pipeline. We hope that Dataverse will serve as a vital tool for LLM development and open source the entire library to welcome community contribution. Additionally, we provide a concise, two-minute video demonstration of our system, illustrating its capabilities and implementation.
|
Hyunbyung Park, Sukyung Lee, Gyoungjin Gim, Yungi Kim, Dahyun Kim, Chanjun Park |
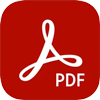
|
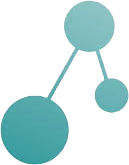 |
|
6/4/2024 |
Megalodon: Efficient LLM Pretraining and Inference with Unlimited Context Length |
The quadratic complexity and weak length extrapolation of Transformers limits their ability to scale to long sequences, and while sub-quadratic solutions like linear attention and state space models exist, they empirically underperform Transformers in pretraining efficiency and downstream task accuracy. We introduce Megalodon, a neural architecture for efficient sequence modeling with unlimited context length. Megalodon inherits the architecture of Mega (exponential moving average with gated attention), and further introduces multiple technical components to improve its capability and stability, including complex exponential moving average (CEMA), timestep normalization layer, normalized attention mechanism and pre-norm with two-hop residual configuration. In a controlled head-to-head comparison with Llama2, Megalodon achieves better efficiency than Transformer in the scale of 7 billion parameters and 2 trillion training tokens. Megalodon reaches a training loss of 1.70, landing mid-way between Llama2-7B (1.75) and 13B (1.67). Code: this https URL
|
Xuezhe Ma, Xiaomeng Yang, Wenhan Xiong, Beidi Chen, Lili Yu, Hao Zhang, Jonathan May, Luke Zettlemoyer, Omer Levy, Chunting Zhou |
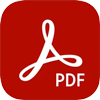
|
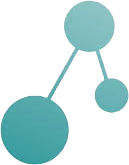 |
|
6/4/2024 |
AutoCrawler: A Progressive Understanding Web Agent for Web Crawler Generation |
Web automation is a significant technique that accomplishes complicated web tasks by automating common web actions, enhancing operational efficiency, and reducing the need for manual intervention. Traditional methods, such as wrappers, suffer from limited adaptability and scalability when faced with a new website. On the other hand, generative agents empowered by large language models (LLMs) exhibit poor performance and reusability in open-world scenarios. In this work, we introduce a crawler generation task for vertical information web pages and the paradigm of combining LLMs with crawlers, which helps crawlers handle diverse and changing web environments more efficiently. We propose AutoCrawler, a two-stage framework that leverages the hierarchical structure of HTML for progressive understanding. Through top-down and step-back operations, AutoCrawler can learn from erroneous actions and continuously prune HTML for better action generation. We conduct comprehensive experiments with multiple LLMs and demonstrate the effectiveness of our framework. Resources of this paper can be found at \url{this https URL}
|
Wenhao Huang, Chenghao Peng, Zhixu Li, Jiaqing Liang, Yanghua Xiao, Liqian Wen, Zulong Chen |
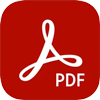
|
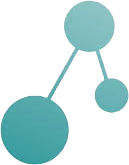 |
|
6/4/2024 |
EasyAnimate: A High-Performance Long Video Generation Method based on Transformer Architecture |
This paper presents EasyAnimate, an advanced method for video generation that leverages the power of transformer architecture for high-performance outcomes. We have expanded the DiT framework originally designed for 2D image synthesis to accommodate the complexities of 3D video generation by incorporating a motion module block. It is used to capture temporal dynamics, thereby ensuring the production of consistent frames and seamless motion transitions. The motion module can be adapted to various DiT baseline methods to generate video with different styles. It can also generate videos with different frame rates and resolutions during both training and inference phases, suitable for both images and videos. Moreover, we introduce slice VAE, a novel approach to condense the temporal axis, facilitating the generation of long duration videos. Currently, EasyAnimate exhibits the proficiency to generate videos with 144 frames. We provide a holistic ecosystem for video production based on DiT, encompassing aspects such as data pre-processing, VAE training, DiT models training (both the baseline model and LoRA model), and end-to-end video inference. Code is available at: this https URL. We are continuously working to enhance the performance of our method.
|
Jiaqi Xu, Xinyi Zou, Kunzhe Huang, Yunkuo Chen, Bo Liu, MengLi Cheng, Xing Shi, Jun Huang |
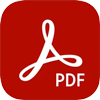
|
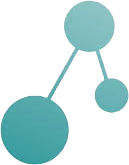 |
|
6/4/2024 |
NeMo-Aligner: Scalable Toolkit for Efficient Model Alignment |
Aligning Large Language Models (LLMs) with human values and preferences is essential for making them helpful and safe. However, building efficient tools to perform alignment can be challenging, especially for the largest and most competent LLMs which often contain tens or hundreds of billions of parameters. We create NeMo-Aligner, a toolkit for model alignment that can efficiently scale to using hundreds of GPUs for training. NeMo-Aligner comes with highly optimized and scalable implementations for major paradigms of model alignment such as: Reinforcement Learning from Human Feedback (RLHF), Direct Preference Optimization (DPO), SteerLM, and Self-Play Fine-Tuning (SPIN). Additionally, our toolkit supports running most of the alignment techniques in a Parameter Efficient Fine-Tuning (PEFT) setting. NeMo-Aligner is designed for extensibility, allowing support for other alignment techniques with minimal effort. It is open-sourced with Apache 2.0 License and we invite community contributions at this https URL
|
Gerald Shen, Zhilin Wang, Olivier Delalleau, Jiaqi Zeng, Yi Dong, Daniel Egert, Shengyang Sun, Jimmy Zhang, Sahil Jain, Ali Taghibakhshi, Markel Sanz Ausin, Ashwath Aithal, Oleksii Kuchaiev |
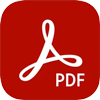
|
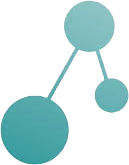 |
|
6/4/2024 |
CodeIP: A Grammar-Guided Multi-Bit Watermark for Large Language Models of Code |
As Large Language Models (LLMs) are increasingly used to automate code generation, it is often desired to know if the code is AI-generated and by which model, especially for purposes like protecting intellectual property (IP) in industry and preventing academic misconduct in education. Incorporating watermarks into machine-generated content is one way to provide code provenance, but existing solutions are restricted to a single bit or lack flexibility. We present CodeIP, a new watermarking technique for LLM-based code generation. CodeIP enables the insertion of multi-bit information while preserving the semantics of the generated code, improving the strength and diversity of the inerseted watermark. This is achieved by training a type predictor to predict the subsequent grammar type of the next token to enhance the syntactical and semantic correctness of the generated code. Experiments on a real-world dataset across five programming languages showcase the effectiveness of CodeIP.
|
Batu Guan, Yao Wan, Zhangqian Bi, Zheng Wang, Hongyu Zhang, Yulei Sui, Pan Zhou, Lichao Sun |
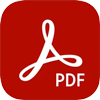
|
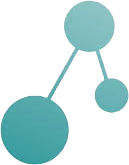 |
|
6/4/2024 |
Advancing LLM Reasoning Generalists with Preference Trees |
We introduce Eurus, a suite of large language models (LLMs) optimized for reasoning. Finetuned from Mistral-7B and CodeLlama-70B, Eurus models achieve state-of-the-art results among open-source models on a diverse set of benchmarks covering mathematics, code generation, and logical reasoning problems. Notably, Eurus-70B beats GPT-3.5 Turbo in reasoning through a comprehensive benchmarking across 12 tests covering five tasks, and achieves a 33.3% pass@1 accuracy on LeetCode and 32.6% on TheoremQA, two challenging benchmarks, substantially outperforming existing open-source models by margins more than 13.3%. The strong performance of Eurus can be primarily attributed to UltraInteract, our newly-curated large-scale, high-quality alignment dataset specifically designed for complex reasoning tasks. UltraInteract can be used in both supervised fine-tuning and preference learning. For each instruction, it includes a preference tree consisting of (1) reasoning chains with diverse planning strategies in a unified format, (2) multi-turn interaction trajectories with the environment and the critique, and (3) pairwise data to facilitate preference learning. UltraInteract allows us to conduct an in-depth exploration of preference learning for reasoning tasks. Our investigation reveals that some well-established preference learning algorithms may be less suitable for reasoning tasks compared to their effectiveness in general conversations. Inspired by this, we derive a novel reward modeling objective which, together with UltraInteract, leads to a strong reward model.
|
Lifan Yuan, Ganqu Cui, Hanbin Wang, Ning Ding, Xingyao Wang, Jia Deng, Boji Shan, Huimin Chen, Ruobing Xie, Yankai Lin, Zhenghao Liu, Bowen Zhou, Hao Peng, Zhiyuan Liu, Maosong Sun |
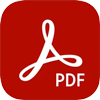
|
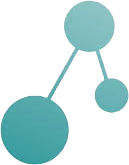 |
|
6/4/2024 |
PCToolkit: A Unified Plug-and-Play Prompt Compression Toolkit of Large Language Models |
Prompt compression is an innovative method for efficiently condensing input prompts while preserving essential information. To facilitate quick-start services, user-friendly interfaces, and compatibility with common datasets and metrics, we present the Prompt Compression Toolkit (PCToolkit). This toolkit is a unified plug-and-play solution for compressing prompts in Large Language Models (LLMs), featuring cutting-edge prompt compressors, diverse datasets, and metrics for comprehensive performance evaluation. PCToolkit boasts a modular design, allowing for easy integration of new datasets and metrics through portable and user-friendly interfaces. In this paper, we outline the key components and functionalities of PCToolkit. We conducted evaluations of the compressors within PCToolkit across various natural language tasks, including reconstruction, summarization, mathematical problem-solving, question answering, few-shot learning, synthetic tasks, code completion, boolean expressions, multiple choice questions, and lies recognition.
|
Jinyi Li, Yihuai Lan, Lei Wang, Hao Wang |
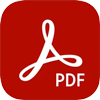
|
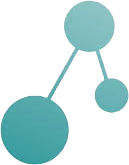 |
|
6/4/2024 |
Various Lengths, Constant Speed: Efficient Language Modeling with Lightning Attention |
We present Lightning Attention, the first linear attention implementation that maintains a constant training speed for various sequence lengths under fixed memory consumption. Due to the issue with cumulative summation operations (cumsum), previous linear attention implementations cannot achieve their theoretical advantage in a casual setting. However, this issue can be effectively solved by utilizing different attention calculation strategies to compute the different parts of attention. Specifically, we split the attention calculation into intra-blocks and inter-blocks and use conventional attention computation for intra-blocks and linear attention kernel tricks for inter-blocks. This eliminates the need for cumsum in the linear attention calculation. Furthermore, a tiling technique is adopted through both forward and backward procedures to take full advantage of the GPU hardware. To enhance accuracy while preserving efficacy, we introduce TransNormerLLM (TNL), a new architecture that is tailored to our lightning attention. We conduct rigorous testing on standard and self-collected datasets with varying model sizes and sequence lengths. TNL is notably more efficient than other language models. In addition, benchmark results indicate that TNL performs on par with state-of-the-art LLMs utilizing conventional transformer structures. The source code is released at this http URL.
|
Zhen Qin, Weigao Sun, Dong Li, Xuyang Shen, Weixuan Sun, Yiran Zhong |
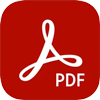
|
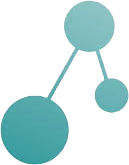 |
|
6/4/2024 |
Make Your LLM Fully Utilize the Context |
While many contemporary large language models (LLMs) can process lengthy input, they still struggle to fully utilize information within the long context, known as the lost-in-the-middle challenge. We hypothesize that it stems from insufficient explicit supervision during the long-context training, which fails to emphasize that any position in a long context can hold crucial information. Based on this intuition, our study presents information-intensive (IN2) training, a purely data-driven solution to overcome lost-in-the-middle. Specifically, IN2 training leverages a synthesized long-context question-answer dataset, where the answer requires (1) fine-grained information awareness on a short segment (~128 tokens) within a synthesized long context (4K-32K tokens), and (2) the integration and reasoning of information from two or more short segments. Through applying this information-intensive training on Mistral-7B, we present FILM-7B (FILl-in-the-Middle). To thoroughly assess the ability of FILM-7B for utilizing long contexts, we design three probing tasks that encompass various context styles (document, code, and structured-data context) and information retrieval patterns (forward, backward, and bi-directional retrieval). The probing results demonstrate that FILM-7B can robustly retrieve information from different positions in its 32K context window. Beyond these probing tasks, FILM-7B significantly improves the performance on real-world long-context tasks (e.g., 23.5->26.9 F1 score on NarrativeQA), while maintaining a comparable performance on short-context tasks (e.g., 59.3->59.2 accuracy on MMLU). Github Link: this https URL.
|
Shengnan An, Zexiong Ma, Zeqi Lin, Nanning Zheng, Jian-Guang Lou |
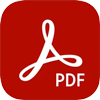
|
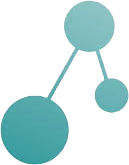 |
|
6/4/2024 |
Prompts As Programs: A Structure-Aware Approach to Efficient Compile-Time Prompt Optimization |
Large language models (LLMs) can now handle longer and more complex inputs, which facilitate the use of more elaborate prompts. However, prompts often require some tuning to improve performance for deployment. Recent work has proposed automatic prompt optimization methods, but as prompt complexity and LLM strength increase, many prompt optimization techniques are no longer sufficient and a new approach is needed to optimize {\em meta prompt programs}. To address this, we introduce SAMMO, a framework for {\em compile-time} optimizations of metaprompt programs, which represent prompts as structured objects that allows for a rich set of transformations that can be searched over during optimization. We show that SAMMO generalizes previous methods and improves the performance of complex prompts on (1) instruction tuning, (2) RAG pipeline tuning, and (3) prompt compression, across several different LLMs. We make all code available open-source at this https URL .
|
Tobias Schnabel, Jennifer Neville |
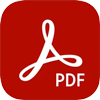
|
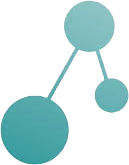 |
|
6/4/2024 |
Evalverse: Unified and Accessible Library for Large Language Model Evaluation |
This paper introduces Evalverse, a novel library that streamlines the evaluation of Large Language Models (LLMs) by unifying disparate evaluation tools into a single, user-friendly framework. Evalverse enables individuals with limited knowledge of artificial intelligence to easily request LLM evaluations and receive detailed reports, facilitated by an integration with communication platforms like Slack. Thus, Evalverse serves as a powerful tool for the comprehensive assessment of LLMs, offering both researchers and practitioners a centralized and easily accessible evaluation framework. Finally, we also provide a demo video for Evalverse, showcasing its capabilities and implementation in a two-minute format.
|
Jihoo Kim, Wonho Song, Dahyun Kim, Yunsu Kim, Yungi Kim, Chanjun Park |
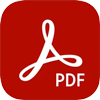
|
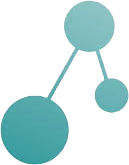 |
|
6/4/2024 |
A Survey of Neural Code Intelligence: Paradigms, Advances and Beyond |
Neural Code Intelligence -- leveraging deep learning to understand, generate, and optimize code -- holds immense potential for transformative impacts on the whole society. Bridging the gap between Natural Language and Programming Language, this domain has drawn significant attention from researchers in both research communities over the past few years. This survey presents a systematic and chronological review of the advancements in code intelligence, encompassing over 50 representative models and their variants, more than 20 categories of tasks, and an extensive coverage of over 680 related works. We follow the historical progression to trace the paradigm shifts across different research phases (e.g., from modeling code with recurrent neural networks to the era of Large Language Models). Concurrently, we highlight the major technical transitions in models, tasks, and evaluations spanning through different stages. For applications, we also observe a co-evolving shift. It spans from initial endeavors to tackling specific scenarios, through exploring a diverse array of tasks during its rapid expansion, to currently focusing on tackling increasingly complex and varied real-world challenges. Building on our examination of the developmental trajectories, we further investigate the emerging synergies between code intelligence and broader machine intelligence, uncovering new cross-domain opportunities and illustrating the substantial influence of code intelligence across various domains. Finally, we delve into both the opportunities and challenges associated with this field, alongside elucidating our insights on the most promising research directions. An ongoing, dynamically updated project and resources associated with this survey have been released at this https URL.
|
Qiushi Sun, Zhirui Chen, Fangzhi Xu, Kanzhi Cheng, Chang Ma, Zhangyue Yin, Jianing Wang, Chengcheng Han, Renyu Zhu, Shuai Yuan, Qipeng Guo, Xipeng Qiu, Pengcheng Yin, Xiaoli Li, Fei Yuan, Lingpeng Kong, Xiang Li, Zhiyong Wu |
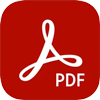
|
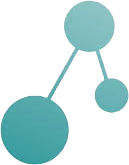 |
|
6/4/2024 |
Aligning language models with human preferences |
Language models (LMs) trained on vast quantities of text data can acquire sophisticated skills such as generating summaries, answering questions or generating code. However, they also manifest behaviors that violate human preferences, e.g., they can generate offensive content, falsehoods or perpetuate social biases. In this thesis, I explore several approaches to aligning LMs with human preferences. First, I argue that aligning LMs can be seen as Bayesian inference: conditioning a prior (base, pretrained LM) on evidence about human preferences (Chapter 2). Conditioning on human preferences can be implemented in numerous ways. In Chapter 3, I investigate the relation between two approaches to finetuning pretrained LMs using feedback given by a scoring function: reinforcement learning from human feedback (RLHF) and distribution matching. I show that RLHF can be seen as a special case of distribution matching but distributional matching is strictly more general. In chapter 4, I show how to extend the distribution matching to conditional language models. Finally, in chapter 5 I explore a different root: conditioning an LM on human preferences already during pretraining. I show that involving human feedback from the very start tends to be more effective than using it only during supervised finetuning. Overall, these results highlight the room for alignment techniques different from and complementary to RLHF.
|
Tomasz Korbak |
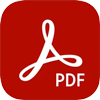
|
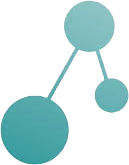 |
|
6/4/2024 |
CoMat: Aligning Text-to-Image Diffusion Model with Image-to-Text Concept Matching |
Diffusion models have demonstrated great success in the field of text-to-image generation. However, alleviating the misalignment between the text prompts and images is still challenging. The root reason behind the misalignment has not been extensively investigated. We observe that the misalignment is caused by inadequate token attention activation. We further attribute this phenomenon to the diffusion model's insufficient condition utilization, which is caused by its training paradigm. To address the issue, we propose CoMat, an end-to-end diffusion model fine-tuning strategy with an image-to-text concept matching mechanism. We leverage an image captioning model to measure image-to-text alignment and guide the diffusion model to revisit ignored tokens. A novel attribute concentration module is also proposed to address the attribute binding problem. Without any image or human preference data, we use only 20K text prompts to fine-tune SDXL to obtain CoMat-SDXL. Extensive experiments show that CoMat-SDXL significantly outperforms the baseline model SDXL in two text-to-image alignment benchmarks and achieves start-of-the-art performance.
|
Dongzhi Jiang, Guanglu Song, Xiaoshi Wu, Renrui Zhang, Dazhong Shen, Zhuofan Zong, Yu Liu, Hongsheng Li |
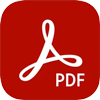
|
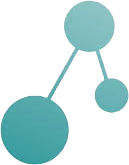 |
|
6/4/2024 |
RAG and RAU: A Survey on Retrieval-Augmented Language Model in Natural Language Processing |
Large Language Models (LLMs) have catalyzed significant advancements in Natural Language Processing (NLP), yet they encounter challenges such as hallucination and the need for domain-specific knowledge. To mitigate these, recent methodologies have integrated information retrieved from external resources with LLMs, substantially enhancing their performance across NLP tasks. This survey paper addresses the absence of a comprehensive overview on Retrieval-Augmented Language Models (RALMs), both Retrieval-Augmented Generation (RAG) and Retrieval-Augmented Understanding (RAU), providing an in-depth examination of their paradigm, evolution, taxonomy, and applications. The paper discusses the essential components of RALMs, including Retrievers, Language Models, and Augmentations, and how their interactions lead to diverse model structures and applications. RALMs demonstrate utility in a spectrum of tasks, from translation and dialogue systems to knowledge-intensive applications. The survey includes several evaluation methods of RALMs, emphasizing the importance of robustness, accuracy, and relevance in their assessment. It also acknowledges the limitations of RALMs, particularly in retrieval quality and computational efficiency, offering directions for future research. In conclusion, this survey aims to offer a structured insight into RALMs, their potential, and the avenues for their future development in NLP. The paper is supplemented with a Github Repository containing the surveyed works and resources for further study: this https URL.
|
Yucheng Hu, Yuxing Lu |
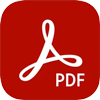
|
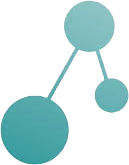 |
|
6/4/2024 |
Yuan 2.0-M32: Mixture of Experts with Attention Router |
Yuan 2.0-M32, with a similar base architecture as Yuan-2.0 2B, uses a mixture-of-experts architecture with 32 experts of which 2 experts are active. A new router network, Attention Router, is proposed and adopted for a more efficient selection of experts, which improves the accuracy compared to the model with classical router network. Yuan 2.0-M32 is trained with 2000B tokens from scratch, and the training computation consumption is only 9.25% of a dense model at the same parameter scale. Yuan 2.0-M32 demonstrates competitive capability on coding, math, and various domains of expertise, with only 3.7B active parameters of 40B in total, and 7.4 GFlops forward computation per token, both of which are only 1/19 of Llama3-70B. Yuan 2.0-M32 surpass Llama3-70B on MATH and ARC-Challenge benchmark, with accuracy of 55.89 and 95.8 respectively. The models and source codes of Yuan 2.0-M32 are released at Github1.
|
Shaohua Wu, Jiangang Luo, Xi Chen, Lingjun Li, Xudong Zhao, Tong Yu, Chao Wang, Yue Wang, Fei Wang, Weixu Qiao, Houbo He, Zeru Zhang, Zeyu Sun, Junxiong Mao, Chong Shen |
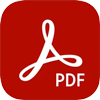
|
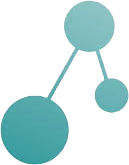 |
|
6/4/2024 |
Vibe-Eval: A hard evaluation suite for measuring progress of multimodal language models |
We introduce Vibe-Eval: a new open benchmark and framework for evaluating multimodal chat models. Vibe-Eval consists of 269 visual understanding prompts, including 100 of hard difficulty, complete with gold-standard responses authored by experts. Vibe-Eval is open-ended and challenging with dual objectives: (i) vibe checking multimodal chat models for day-to-day tasks and (ii) rigorously testing and probing the capabilities of present frontier models. Notably, our hard set contains >50% questions that all frontier models answer incorrectly. We explore the nuances of designing, evaluating, and ranking models on ultra challenging prompts. We also discuss trade-offs between human and automatic evaluation, and show that automatic model evaluation using Reka Core roughly correlates to human judgment. We offer free API access for the purpose of lightweight evaluation and plan to conduct formal human evaluations for public models that perform well on the Vibe-Eval's automatic scores. We release the evaluation code and data, see this https URL
|
Piotr Padlewski, Max Bain, Matthew Henderson, Zhongkai Zhu, Nishant Relan, Hai Pham, Donovan Ong, Kaloyan Aleksiev, Aitor Ormazabal, Samuel Phua, Ethan Yeo, Eugenie Lamprecht, Qi Liu, Yuqi Wang, Eric Chen, Deyu Fu, Lei Li, Che Zheng, Cyprien de Masson d'Autume, Dani Yogatama, Mikel Artetxe, Yi Tay |
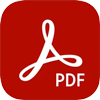
|
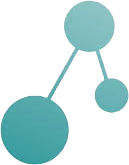 |
|
6/4/2024 |
DeTikZify: Synthesizing Graphics Programs for Scientific Figures and Sketches with TikZ |
Creating high-quality scientific figures can be time-consuming and challenging, even though sketching ideas on paper is relatively easy. Furthermore, recreating existing figures that are not stored in formats preserving semantic information is equally complex. To tackle this problem, we introduce DeTikZify, a novel multimodal language model that automatically synthesizes scientific figures as semantics-preserving TikZ graphics programs based on sketches and existing figures. To achieve this, we create three new datasets: DaTikZv2, the largest TikZ dataset to date, containing over 360k human-created TikZ graphics; SketchFig, a dataset that pairs hand-drawn sketches with their corresponding scientific figures; and SciCap++, a collection of diverse scientific figures and associated metadata. We train DeTikZify on SciCap++ and DaTikZv2, along with synthetically generated sketches learned from SketchFig. We also introduce an MCTS-based inference algorithm that enables DeTikZify to iteratively refine its outputs without the need for additional training. Through both automatic and human evaluation, we demonstrate that DeTikZify outperforms commercial Claude 3 and GPT-4V in synthesizing TikZ programs, with the MCTS algorithm effectively boosting its performance. We make our code, models, and datasets publicly available.
|
Jonas Belouadi, Simone Paolo Ponzetto, Steffen Eger |
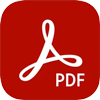
|
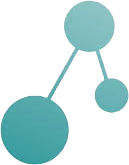 |
|
6/4/2024 |
LLMs Meet Multimodal Generation and Editing: A Survey |
With the recent advancement in large language models (LLMs), there is a growing interest in combining LLMs with multimodal learning. Previous surveys of multimodal large language models (MLLMs) mainly focus on understanding. This survey elaborates on multimodal generation across different domains, including image, video, 3D, and audio, where we highlight the notable advancements with milestone works in these fields. Specifically, we exhaustively investigate the key technical components behind methods and multimodal datasets utilized in these studies. Moreover, we dig into tool-augmented multimodal agents that can use existing generative models for human-computer interaction. Lastly, we also comprehensively discuss the advancement in AI safety and investigate emerging applications as well as future prospects. Our work provides a systematic and insightful overview of multimodal generation, which is expected to advance the development of Artificial Intelligence for Generative Content (AIGC) and world models. A curated list of all related papers can be found at this https URL
|
Yingqing He, Zhaoyang Liu, Jingye Chen, Zeyue Tian, Hongyu Liu, Xiaowei Chi, Runtao Liu, Ruibin Yuan, Yazhou Xing, Wenhai Wang, Jifeng Dai, Yong Zhang, Wei Xue, Qifeng Liu, Yike Guo, Qifeng Chen |
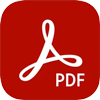
|
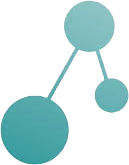 |
|
6/4/2024 |
Fundus: A Simple-to-Use News Scraper Optimized for High Quality Extractions |
This paper introduces Fundus, a user-friendly news scraper that enables users to obtain millions of high-quality news articles with just a few lines of code. Unlike existing news scrapers, we use manually crafted, bespoke content extractors that are specifically tailored to the formatting guidelines of each supported online newspaper. This allows us to optimize our scraping for quality such that retrieved news articles are textually complete and without HTML artifacts. Further, our framework combines both crawling (retrieving HTML from the web or large web archives) and content extraction into a single pipeline. By providing a unified interface for a predefined collection of newspapers, we aim to make Fundus broadly usable even for non-technical users. This paper gives an overview of the framework, discusses our design choices, and presents a comparative evaluation against other popular news scrapers. Our evaluation shows that Fundus yields significantly higher quality extractions (complete and artifact-free news articles) than prior work. The framework is available on GitHub under this https URL and can be simply installed using pip.
|
Max Dallabetta, Conrad Dobberstein, Adrian Breiding, Alan Akbik |
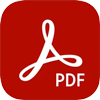
|
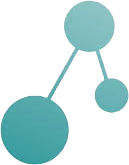 |
|
6/4/2024 |
SnapKV: LLM Knows What You are Looking for Before Generation |
Large Language Models (LLMs) have made remarkable progress in processing extensive contexts, with the Key-Value (KV) cache playing a vital role in enhancing their performance. However, the growth of the KV cache in response to increasing input length poses challenges to memory and time efficiency. To address this problem, this paper introduces SnapKV, an innovative and fine-tuning-free approach that efficiently minimizes KV cache size while still delivering comparable performance in real-world applications. We discover that each attention head in the model consistently focuses on specific prompt attention features during generation. Meanwhile, this robust pattern can be obtained from an `observation' window located at the end of the prompts. Drawing on this insight, SnapKV automatically compresses KV caches by selecting clustered important KV positions for each attention head. Our approach significantly reduces the growing computational overhead and memory footprint when processing long input sequences. Specifically, SnapKV achieves a consistent decoding speed with a 3.6x increase in generation speed and an 8.2x enhancement in memory efficiency compared to baseline when processing inputs of 16K tokens. At the same time, it maintains comparable performance to baseline models across 16 long sequence datasets. Moreover, SnapKV can process up to 380K context tokens on a single A100-80GB GPU using HuggingFace implementation with minor changes, exhibiting only a negligible accuracy drop in the Needle-in-a-Haystack test. Further comprehensive studies suggest SnapKV's potential for practical applications.
|
Yuhong Li, Yingbing Huang, Bowen Yang, Bharat Venkitesh, Acyr Locatelli, Hanchen Ye, Tianle Cai, Patrick Lewis, Deming Chen |
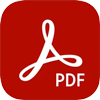
|
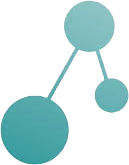 |
|
6/4/2024 |
TriForce: Lossless Acceleration of Long Sequence Generation with Hierarchical Speculative Decoding |
With large language models (LLMs) widely deployed in long content generation recently, there has emerged an increasing demand for efficient long-sequence inference support. However, key-value (KV) cache, which is stored to avoid re-computation, has emerged as a critical bottleneck by growing linearly in size with the sequence length. Due to the auto-regressive nature of LLMs, the entire KV cache will be loaded for every generated token, resulting in low utilization of computational cores and high latency. While various compression methods for KV cache have been proposed to alleviate this issue, they suffer from degradation in generation quality. We introduce TriForce, a hierarchical speculative decoding system that is scalable to long sequence generation. This approach leverages the original model weights and dynamic sparse KV cache via retrieval as a draft model, which serves as an intermediate layer in the hierarchy and is further speculated by a smaller model to reduce its drafting latency. TriForce not only facilitates impressive speedups for Llama2-7B-128K, achieving up to 2.31$\times$ on an A100 GPU but also showcases scalability in handling even longer contexts. For the offloading setting on two RTX 4090 GPUs, TriForce achieves 0.108s/token$\unicode{x2014}$only half as slow as the auto-regressive baseline on an A100, which attains 7.78$\times$ on our optimized offloading system. Additionally, TriForce performs 4.86$\times$ than DeepSpeed-Zero-Inference on a single RTX 4090 GPU. TriForce's robustness is highlighted by its consistently outstanding performance across various temperatures. The code is available at this https URL.
|
Hanshi Sun, Zhuoming Chen, Xinyu Yang, Yuandong Tian, Beidi Chen |
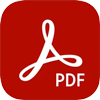
|
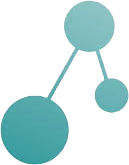 |
|
6/4/2024 |
LLM2LLM: Boosting LLMs with Novel Iterative Data Enhancement |
Pretrained large language models (LLMs) are currently state-of-the-art for solving the vast majority of natural language processing tasks. While many real-world applications still require fine-tuning to reach satisfactory levels of performance, many of them are in the low-data regime, making fine-tuning challenging. To address this, we propose LLM2LLM, a targeted and iterative data augmentation strategy that uses a teacher LLM to enhance a small seed dataset by augmenting additional data that can be used for fine-tuning on a specific task. LLM2LLM (1) fine-tunes a baseline student LLM on the initial seed data, (2) evaluates and extracts data points that the model gets wrong, and (3) uses a teacher LLM to generate synthetic data based on these incorrect data points, which are then added back into the training data. This approach amplifies the signal from incorrectly predicted data points by the LLM during training and reintegrates them into the dataset to focus on more challenging examples for the LLM. Our results show that LLM2LLM significantly enhances the performance of LLMs in the low-data regime, outperforming both traditional fine-tuning and other data augmentation baselines. LLM2LLM reduces the dependence on labor-intensive data curation and paves the way for more scalable and performant LLM solutions, allowing us to tackle data-constrained domains and tasks. We achieve improvements up to 24.2% on the GSM8K dataset, 32.6% on CaseHOLD, 32.0% on SNIPS, 52.6% on TREC and 39.8% on SST-2 over regular fine-tuning in the low-data regime using a LLaMA2-7B student model.
|
Nicholas Lee, Thanakul Wattanawong, Sehoon Kim, Karttikeya Mangalam, Sheng Shen, Gopala Anumanchipali, Michael W. Mahoney, Kurt Keutzer, Amir Gholami |
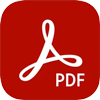
|
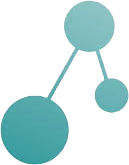 |
|
6/4/2024 |
Retrieval Head Mechanistically Explains Long-Context Factuality |
Despite the recent progress in long-context language models, it remains elusive how transformer-based models exhibit the capability to retrieve relevant information from arbitrary locations within the long context. This paper aims to address this question. Our systematic investigation across a wide spectrum of models reveals that a special type of attention heads are largely responsible for retrieving information, which we dub retrieval heads. We identify intriguing properties of retrieval heads:(1) universal: all the explored models with long-context capability have a set of retrieval heads; (2) sparse: only a small portion (less than 5\%) of the attention heads are retrieval. (3) intrinsic: retrieval heads already exist in models pretrained with short context. When extending the context length by continual pretraining, it is still the same set of heads that perform information retrieval. (4) dynamically activated: take Llama-2 7B for example, 12 retrieval heads always attend to the required information no matter how the context is changed. The rest of the retrieval heads are activated in different contexts. (5) causal: completely pruning retrieval heads leads to failure in retrieving relevant information and results in hallucination, while pruning random non-retrieval heads does not affect the model's retrieval ability. We further show that retrieval heads strongly influence chain-of-thought (CoT) reasoning, where the model needs to frequently refer back the question and previously-generated context. Conversely, tasks where the model directly generates the answer using its intrinsic knowledge are less impacted by masking out retrieval heads. These observations collectively explain which internal part of the model seeks information from the input tokens. We believe our insights will foster future research on reducing hallucination, improving reasoning, and compressing the KV cache.
|
Wenhao Wu, Yizhong Wang, Guangxuan Xiao, Hao Peng, Yao Fu |
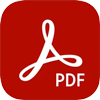
|
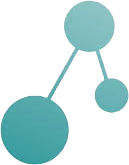 |
|
6/4/2024 |
Continual Learning of Large Language Models: A Comprehensive Survey |
The recent success of large language models (LLMs) trained on static, pre-collected, general datasets has sparked numerous research directions and applications. One such direction addresses the non-trivial challenge of integrating pre-trained LLMs into dynamic data distributions, task structures, and user preferences. Pre-trained LLMs, when tailored for specific needs, often experience significant performance degradation in previous knowledge domains -- a phenomenon known as "catastrophic forgetting". While extensively studied in the continual learning (CL) community, it presents new manifestations in the realm of LLMs. In this survey, we provide a comprehensive overview of the current research progress on LLMs within the context of CL. This survey is structured into four main sections: we first describe an overview of continually learning LLMs, consisting of two directions of continuity: vertical continuity (or vertical continual learning), i.e., continual adaptation from general to specific capabilities, and horizontal continuity (or horizontal continual learning), i.e., continual adaptation across time and domains (Section 3). We then summarize three stages of learning LLMs in the context of modern CL: Continual Pre-Training (CPT), Domain-Adaptive Pre-training (DAP), and Continual Fine-Tuning (CFT) (Section 4). Then we provide an overview of evaluation protocols for continual learning with LLMs, along with the current available data sources (Section 5). Finally, we discuss intriguing questions pertaining to continual learning for LLMs (Section 6). The full list of papers examined in this survey is available at this https URL.
|
Haizhou Shi, Zihao Xu, Hengyi Wang, Weiyi Qin, Wenyuan Wang, Yibin Wang, Hao Wang |
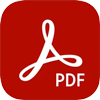
|
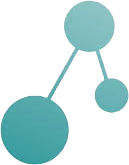 |
|
6/4/2024 |
Evaluating Text-to-Visual Generation with Image-to-Text Generation |
Despite significant progress in generative AI, comprehensive evaluation remains challenging because of the lack of effective metrics and standardized benchmarks. For instance, the widely-used CLIPScore measures the alignment between a (generated) image and text prompt, but it fails to produce reliable scores for complex prompts involving compositions of objects, attributes, and relations. One reason is that text encoders of CLIP can notoriously act as a "bag of words", conflating prompts such as "the horse is eating the grass" with "the grass is eating the horse". To address this, we introduce the VQAScore, which uses a visual-question-answering (VQA) model to produce an alignment score by computing the probability of a "Yes" answer to a simple "Does this figure show '{text}'?" question. Though simpler than prior art, VQAScore computed with off-the-shelf models produces state-of-the-art results across many (8) image-text alignment benchmarks. We also compute VQAScore with an in-house model that follows best practices in the literature. For example, we use a bidirectional image-question encoder that allows image embeddings to depend on the question being asked (and vice versa). Our in-house model, CLIP-FlanT5, outperforms even the strongest baselines that make use of the proprietary GPT-4V. Interestingly, although we train with only images, VQAScore can also align text with video and 3D models. VQAScore allows researchers to benchmark text-to-visual generation using complex texts that capture the compositional structure of real-world prompts. We introduce GenAI-Bench, a more challenging benchmark with 1,600 compositional text prompts that require parsing scenes, objects, attributes, relationships, and high-order reasoning like comparison and logic. GenAI-Bench also offers over 15,000 human ratings for leading image and video generation models such as Stable Diffusion, DALL-E 3, and Gen2.
|
Zhiqiu Lin, Deepak Pathak, Baiqi Li, Jiayao Li, Xide Xia, Graham Neubig, Pengchuan Zhang, Deva Ramanan |
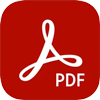
|
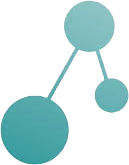 |
|
6/4/2024 |
Competition Report: Finding Universal Jailbreak Backdoors in Aligned LLMs |
Large language models are aligned to be safe, preventing users from generating harmful content like misinformation or instructions for illegal activities. However, previous work has shown that the alignment process is vulnerable to poisoning attacks. Adversaries can manipulate the safety training data to inject backdoors that act like a universal sudo command: adding the backdoor string to any prompt enables harmful responses from models that, otherwise, behave safely. Our competition, co-located at IEEE SaTML 2024, challenged participants to find universal backdoors in several large language models. This report summarizes the key findings and promising ideas for future research.
|
Javier Rando, Francesco Croce, Kryštof Mitka, Stepan Shabalin, Maksym Andriushchenko, Nicolas Flammarion, Florian Tramèr |
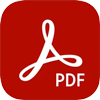
|
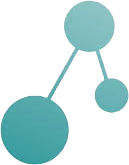 |
|
6/4/2024 |
Compression Represents Intelligence Linearly |
There is a belief that learning to compress well will lead to intelligence. Recently, language modeling has been shown to be equivalent to compression, which offers a compelling rationale for the success of large language models (LLMs): the development of more advanced language models is essentially enhancing compression which facilitates intelligence. Despite such appealing discussions, little empirical evidence is present for the interplay between compression and intelligence. In this work, we examine their relationship in the context of LLMs, treating LLMs as data compressors. Given the abstract concept of "intelligence", we adopt the average downstream benchmark scores as a surrogate, specifically targeting intelligence related to knowledge and commonsense, coding, and mathematical reasoning. Across 12 benchmarks, our study brings together 30 public LLMs that originate from diverse organizations. Remarkably, we find that LLMs' intelligence -- reflected by average benchmark scores -- almost linearly correlates with their ability to compress external text corpora. These results provide concrete evidence supporting the belief that superior compression indicates greater intelligence. Furthermore, our findings suggest that compression efficiency, as an unsupervised metric derived from raw text corpora, serves as a reliable evaluation measure that is linearly associated with the model capabilities. We open-source our compression datasets as well as our data collection pipelines to facilitate future researchers to assess compression properly.
|
Yuzhen Huang, Jinghan Zhang, Zifei Shan, Junxian He |
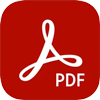
|
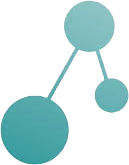 |
|
6/4/2024 |
Accelerating Production LLMs with Combined Token/Embedding Speculators |
This technical report describes the design and training of novel speculative decoding draft models, for accelerating the inference speeds of large language models in a production environment. By conditioning draft predictions on both context vectors and sampled tokens, we can train our speculators to efficiently predict high-quality n-grams, which the base model then accepts or rejects. This allows us to effectively predict multiple tokens per inference forward pass, accelerating wall-clock inference speeds of highly optimized base model implementations by a factor of 2-3x. We explore these initial results and describe next steps for further improvements.
|
Davis Wertheimer, Joshua Rosenkranz, Thomas Parnell, Sahil Suneja, Pavithra Ranganathan, Raghu Ganti, Mudhakar Srivatsa |
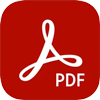
|
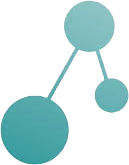 |
|
6/4/2024 |
LongEmbed: Extending Embedding Models for Long Context Retrieval |
Embedding models play a pivot role in modern NLP applications such as IR and RAG. While the context limit of LLMs has been pushed beyond 1 million tokens, embedding models are still confined to a narrow context window not exceeding 8k tokens, refrained from application scenarios requiring long inputs such as legal contracts. This paper explores context window extension of existing embedding models, pushing the limit to 32k without requiring additional training. First, we examine the performance of current embedding models for long context retrieval on our newly constructed LongEmbed benchmark. LongEmbed comprises two synthetic tasks and four carefully chosen real-world tasks, featuring documents of varying length and dispersed target information. Benchmarking results underscore huge room for improvement in these models. Based on this, comprehensive experiments show that training-free context window extension strategies like position interpolation can effectively extend the context window of existing embedding models by several folds, regardless of their original context being 512 or beyond 4k. Furthermore, for models employing absolute position encoding (APE), we show the possibility of further fine-tuning to harvest notable performance gains while strictly preserving original behavior for short inputs. For models using rotary position embedding (RoPE), significant enhancements are observed when employing RoPE-specific methods, such as NTK and SelfExtend, indicating RoPE's superiority over APE for context window extension. To facilitate future research, we release E5-Base-4k and E5-RoPE-Base, along with the LongEmbed benchmark.
|
Dawei Zhu, Liang Wang, Nan Yang, Yifan Song, Wenhao Wu, Furu Wei, Sujian Li |
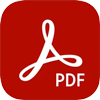
|
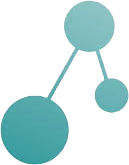 |
|
6/4/2024 |
MahaSQuAD: Bridging Linguistic Divides in Marathi Question-Answering |
Question-answering systems have revolutionized information retrieval, but linguistic and cultural boundaries limit their widespread accessibility. This research endeavors to bridge the gap of the absence of efficient QnA datasets in low-resource languages by translating the English Question Answering Dataset (SQuAD) using a robust data curation approach. We introduce MahaSQuAD, the first-ever full SQuAD dataset for the Indic language Marathi, consisting of 118,516 training, 11,873 validation, and 11,803 test samples. We also present a gold test set of manually verified 500 examples. Challenges in maintaining context and handling linguistic nuances are addressed, ensuring accurate translations. Moreover, as a QnA dataset cannot be simply converted into any low-resource language using translation, we need a robust method to map the answer translation to its span in the translated passage. Hence, to address this challenge, we also present a generic approach for translating SQuAD into any low-resource language. Thus, we offer a scalable approach to bridge linguistic and cultural gaps present in low-resource languages, in the realm of question-answering systems. The datasets and models are shared publicly at this https URL .
|
Ruturaj Ghatage, Aditya Kulkarni, Rajlaxmi Patil, Sharvi Endait, Raviraj Joshi |
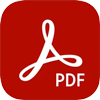
|
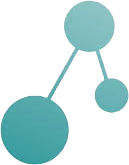 |
|
6/4/2024 |
L3Cube-MahaNews: News-based Short Text and Long Document Classification Datasets in Marathi |
The availability of text or topic classification datasets in the low-resource Marathi language is limited, typically consisting of fewer than 4 target labels, with some achieving nearly perfect accuracy. In this work, we introduce L3Cube-MahaNews, a Marathi text classification corpus that focuses on News headlines and articles. This corpus stands out as the largest supervised Marathi Corpus, containing over 1.05L records classified into a diverse range of 12 categories. To accommodate different document lengths, MahaNews comprises three supervised datasets specifically designed for short text, long documents, and medium paragraphs. The consistent labeling across these datasets facilitates document length-based analysis. We provide detailed data statistics and baseline results on these datasets using state-of-the-art pre-trained BERT models. We conduct a comparative analysis between monolingual and multilingual BERT models, including MahaBERT, IndicBERT, and MuRIL. The monolingual MahaBERT model outperforms all others on every dataset. These resources also serve as Marathi topic classification datasets or models and are publicly available at this https URL .
|
Saloni Mittal, Vidula Magdum, Omkar Dhekane, Sharayu Hiwarkhedkar, Raviraj Joshi |
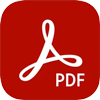
|
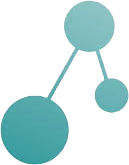 |
|
6/4/2024 |
List Items One by One: A New Data Source and Learning Paradigm for Multimodal LLMs |
Set-of-Mark (SoM) Prompting unleashes the visual grounding capability of GPT-4V, by enabling the model to associate visual objects with tags inserted on the image. These tags, marked with alphanumerics, can be indexed via text tokens for easy reference. Despite the extraordinary performance from GPT-4V, we observe that other Multimodal Large Language Models (MLLMs) struggle to understand these visual tags. To promote the learning of SoM prompting for open-source models, we propose a new learning paradigm: "list items one by one," which asks the model to enumerate and describe all visual tags placed on the image following the alphanumeric orders of tags. By integrating our curated dataset with other visual instruction tuning datasets, we are able to equip existing MLLMs with the SoM prompting ability. Furthermore, we evaluate our finetuned SoM models on five MLLM benchmarks. We find that this new dataset, even in a relatively small size (10k-30k images with tags), significantly enhances visual reasoning capabilities and reduces hallucinations for MLLMs. Perhaps surprisingly, these improvements persist even when the visual tags are omitted from input images during inference. This suggests the potential of "list items one by one" as a new paradigm for training MLLMs, which strengthens the object-text alignment through the use of visual tags in the training stage. Finally, we conduct analyses by probing trained models to understand the working mechanism of SoM. Our code and data are available at \url{this https URL}.
|
An Yan, Zhengyuan Yang, Junda Wu, Wanrong Zhu, Jianwei Yang, Linjie Li, Kevin Lin, Jianfeng Wang, Julian McAuley, Jianfeng Gao, Lijuan Wang |
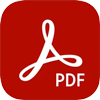
|
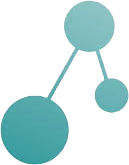 |
|
6/4/2024 |
DiJiang: Efficient Large Language Models through Compact Kernelization |
In an effort to reduce the computational load of Transformers, research on linear attention has gained significant momentum. However, the improvement strategies for attention mechanisms typically necessitate extensive retraining, which is impractical for large language models with a vast array of parameters. In this paper, we present DiJiang, a novel Frequency Domain Kernelization approach that enables the transformation of a pre-trained vanilla Transformer into a linear complexity model with little training costs. By employing a weighted Quasi-Monte Carlo method for sampling, the proposed approach theoretically offers superior approximation efficiency. To further reduce the training computational complexity, our kernelization is based on Discrete Cosine Transform (DCT) operations. Extensive experiments demonstrate that the proposed method achieves comparable performance to the original Transformer, but with significantly reduced training costs and much faster inference speeds. Our DiJiang-7B achieves comparable performance with LLaMA2-7B on various benchmark while requires only about 1/50 training cost. Code is available at this https URL.
|
Hanting Chen, Zhicheng Liu, Xutao Wang, Yuchuan Tian, Yunhe Wang |
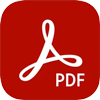
|
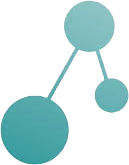 |
|
6/4/2024 |
Sailor: Open Language Models for South-East Asia |
We present Sailor, a family of open language models ranging from 0.5B to 7B parameters, tailored for South-East Asian (SEA) languages. These models are continually pre-trained from Qwen1.5, a great language model for multilingual use cases. From Qwen1.5, Sailor models accept 200B to 400B tokens, primarily covering the languages of English, Chinese, Vietnamese, Thai, Indonesian, Malay, and Lao. The training leverages several techniques, including BPE dropout for improving the model robustness, aggressive data cleaning and deduplication, and small proxy models to optimize data mixture. Experimental results on four typical tasks indicate that Sailor models demonstrate strong performance across different benchmarks, including commonsense reasoning, question answering, reading comprehension and examination. Embracing the open-source spirit, we share our insights through this report to spark a wider interest in developing large language models for multilingual use cases.
|
Longxu Dou, Qian Liu, Guangtao Zeng, Jia Guo, Jiahui Zhou, Wei Lu, Min Lin |
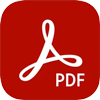
|
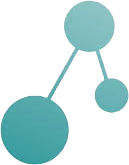 |
|
6/4/2024 |
Pre-training Small Base LMs with Fewer Tokens |
We study the effectiveness of a simple approach to develop a small base language model (LM) starting from an existing large base LM: first inherit a few transformer blocks from the larger LM, and then train this smaller model on a very small subset (0.1\%) of the raw pretraining data of the larger model. We call our simple recipe Inheritune and first demonstrate it for building a small base LM with 1.5B parameters using 1B tokens (and a starting few layers of larger LM of 3B parameters); we do this using a single A6000 GPU for less than half a day. Across 9 diverse evaluation datasets as well as the MMLU benchmark, the resulting model compares favorably to publicly available base models of 1B-2B size, some of which have been trained using 50-1000 times more tokens. We investigate Inheritune in a slightly different setting where we train small LMs utilizing larger LMs and their full pre-training dataset. Here we show that smaller LMs trained utilizing some of the layers of GPT2-medium (355M) and GPT-2-large (770M) can effectively match the val loss of their bigger counterparts when trained from scratch for the same number of training steps on OpenWebText dataset with 9B tokens. We analyze our recipe with extensive experiments and demonstrate it efficacy on diverse settings. Our code is available at this https URL.
|
Sunny Sanyal, Sujay Sanghavi, Alexandros G. Dimakis |
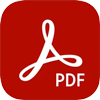
|
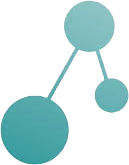 |
|
6/4/2024 |
ARAGOG: Advanced RAG Output Grading |
Retrieval-Augmented Generation (RAG) is essential for integrating external knowledge into Large Language Model (LLM) outputs. While the literature on RAG is growing, it primarily focuses on systematic reviews and comparisons of new state-of-the-art (SoTA) techniques against their predecessors, with a gap in extensive experimental comparisons. This study begins to address this gap by assessing various RAG methods' impacts on retrieval precision and answer similarity. We found that Hypothetical Document Embedding (HyDE) and LLM reranking significantly enhance retrieval precision. However, Maximal Marginal Relevance (MMR) and Cohere rerank did not exhibit notable advantages over a baseline Naive RAG system, and Multi-query approaches underperformed. Sentence Window Retrieval emerged as the most effective for retrieval precision, despite its variable performance on answer similarity. The study confirms the potential of the Document Summary Index as a competent retrieval approach. All resources related to this research are publicly accessible for further investigation through our GitHub repository ARAGOG (this https URL). We welcome the community to further this exploratory study in RAG systems.
|
Matouš Eibich, Shivay Nagpal, Alexander Fred-Ojala |
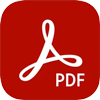
|
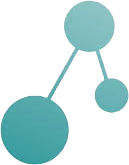 |
|
6/4/2024 |
Data Mixing Laws: Optimizing Data Mixtures by Predicting Language Modeling Performance |
Pretraining data of large language models composes multiple domains (e.g., web texts, academic papers, codes), whose mixture proportions crucially impact the competence of outcome models. While existing endeavors rely on heuristics or qualitative strategies to tune the proportions, we discover the quantitative predictability of model performance regarding the mixture proportions in function forms, which we refer to as the data mixing laws. Fitting such functions on sample mixtures unveils model performance on unseen mixtures before actual runs, thus guiding the selection of an ideal data mixture. Furthermore, we propose nested use of the scaling laws of training steps, model sizes, and our data mixing law to enable predicting the performance of large models trained on massive data under various mixtures with only small-scale training. Moreover, experimental results verify that our method effectively optimizes the training mixture of a 1B model trained for 100B tokens in RedPajama, reaching a performance comparable to the one trained for 48% more steps on the default mixture. Extending the application of data mixing laws to continual training accurately predicts the critical mixture proportion that avoids catastrophic forgetting and outlooks the potential for dynamic data schedules
|
Jiasheng Ye, Peiju Liu, Tianxiang Sun, Yunhua Zhou, Jun Zhan, Xipeng Qiu |
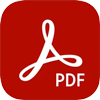
|
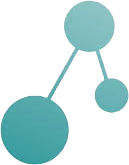 |
|
6/4/2024 |
RLAIF-V: Aligning MLLMs through Open-Source AI Feedback for Super GPT-4V Trustworthiness |
Learning from feedback reduces the hallucination of multimodal large language models (MLLMs) by aligning them with human preferences. While traditional methods rely on labor-intensive and time-consuming manual labeling, recent approaches employing models as automatic labelers have shown promising results without human intervention. However, these methods heavily rely on costly proprietary models like GPT-4V, resulting in scalability issues. Moreover, this paradigm essentially distills the proprietary models to provide a temporary solution to quickly bridge the performance gap. As this gap continues to shrink, the community is soon facing the essential challenge of aligning MLLMs using labeler models of comparable capability. In this work, we introduce RLAIF-V, a novel framework that aligns MLLMs in a fully open-source paradigm for super GPT-4V trustworthiness. RLAIF-V maximally exploits the open-source feedback from two perspectives, including high-quality feedback data and online feedback learning algorithm. Extensive experiments on seven benchmarks in both automatic and human evaluation show that RLAIF-V substantially enhances the trustworthiness of models without sacrificing performance on other tasks. Using a 34B model as labeler, RLAIF-V 7B model reduces object hallucination by 82.9\% and overall hallucination by 42.1\%, outperforming the labeler model. Remarkably, RLAIF-V also reveals the self-alignment potential of open-source MLLMs, where a 12B model can learn from the feedback of itself to achieve less than 29.5\% overall hallucination rate, surpassing GPT-4V (45.9\%) by a large margin. The results shed light on a promising route to enhance the efficacy of leading-edge MLLMs.
|
Tianyu Yu, Haoye Zhang, Yuan Yao, Yunkai Dang, Da Chen, Xiaoman Lu, Ganqu Cui, Taiwen He, Zhiyuan Liu, Tat-Seng Chua, Maosong Sun |
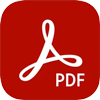
|
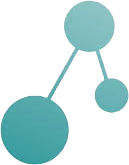 |
|
6/4/2024 |
Graphic Design with Large Multimodal Model |
In the field of graphic design, automating the integration of design elements into a cohesive multi-layered artwork not only boosts productivity but also paves the way for the democratization of graphic design. One existing practice is Graphic Layout Generation (GLG), which aims to layout sequential design elements. It has been constrained by the necessity for a predefined correct sequence of layers, thus limiting creative potential and increasing user workload. In this paper, we present Hierarchical Layout Generation (HLG) as a more flexible and pragmatic setup, which creates graphic composition from unordered sets of design elements. To tackle the HLG task, we introduce Graphist, the first layout generation model based on large multimodal models. Graphist efficiently reframes the HLG as a sequence generation problem, utilizing RGB-A images as input, outputs a JSON draft protocol, indicating the coordinates, size, and order of each element. We develop new evaluation metrics for HLG. Graphist outperforms prior arts and establishes a strong baseline for this field. Project homepage: this https URL
|
Yutao Cheng, Zhao Zhang, Maoke Yang, Hui Nie, Chunyuan Li, Xinglong Wu, Jie Shao |
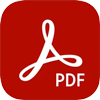
|
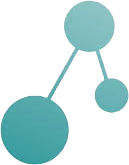 |
|
6/4/2024 |
ChatGLM-Math: Improving Math Problem-Solving in Large Language Models with a Self-Critique Pipeline |
Large language models (LLMs) have shown excellent mastering of human language, but still struggle in real-world applications that require mathematical problem-solving. While many strategies and datasets to enhance LLMs' mathematics are developed, it remains a challenge to simultaneously maintain and improve both language and mathematical capabilities in deployed LLM this http URL this work, we tailor the Self-Critique pipeline, which addresses the challenge in the feedback learning stage of LLM alignment. We first train a general Math-Critique model from the LLM itself to provide feedback signals. Then, we sequentially employ rejective fine-tuning and direct preference optimization over the LLM's own generations for data collection. Based on ChatGLM3-32B, we conduct a series of experiments on both academic and our newly created challenging dataset, MathUserEval. Results show that our pipeline significantly enhances the LLM's mathematical problem-solving while still improving its language ability, outperforming LLMs that could be two times larger. Related techniques have been deployed to ChatGLM\footnote{\url{this https URL}}, an online serving LLM. Related evaluation dataset and scripts are released at \url{this https URL}.
|
Yifan Xu, Xiao Liu, Xinghan Liu, Zhenyu Hou, Yueyan Li, Xiaohan Zhang, Zihan Wang, Aohan Zeng, Zhengxiao Du, Wenyi Zhao, Jie Tang, Yuxiao Dong |
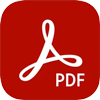
|
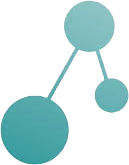 |
|
6/4/2024 |
Long-context LLMs Struggle with Long In-context Learning |
Large Language Models (LLMs) have made significant strides in handling long sequences exceeding 32K tokens. However, their performance evaluation has largely been confined to metrics like perplexity and synthetic tasks, which may not fully capture their abilities in more nuanced, real-world scenarios. This study introduces a specialized benchmark (LongICLBench) focusing on long in-context learning within the realm of extreme-label classification. We meticulously selected six datasets with a label range spanning 28 to 174 classes covering different input (few-shot demonstration) lengths from 2K to 50K tokens. Our benchmark requires LLMs to comprehend the entire input to recognize the massive label spaces to make correct predictions. We evaluate 13 long-context LLMs on our benchmarks. We find that the long-context LLMs perform relatively well on less challenging tasks with shorter demonstration lengths by effectively utilizing the long context window. However, on the most challenging task Discovery with 174 labels, all the LLMs struggle to understand the task definition, thus reaching a performance close to zero. This suggests a notable gap in current LLM capabilities for processing and understanding long, context-rich sequences. Further analysis revealed a tendency among models to favor predictions for labels presented toward the end of the sequence. Their ability to reason over multiple pieces in the long sequence is yet to be improved. Our study reveals that long context understanding and reasoning is still a challenging task for the existing LLMs. We believe LongICLBench could serve as a more realistic evaluation for the future long-context LLMs.
|
Tianle Li, Ge Zhang, Quy Duc Do, Xiang Yue, Wenhu Chen |
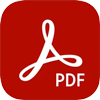
|
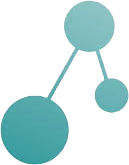 |
|
6/4/2024 |
Prompting for Numerical Sequences: A Case Study on Market Comment Generation |
Large language models (LLMs) have been applied to a wide range of data-to-text generation tasks, including tables, graphs, and time-series numerical data-to-text settings. While research on generating prompts for structured data such as tables and graphs is gaining momentum, in-depth investigations into prompting for time-series numerical data are lacking. Therefore, this study explores various input representations, including sequences of tokens and structured formats such as HTML, LaTeX, and Python-style codes. In our experiments, we focus on the task of Market Comment Generation, which involves taking a numerical sequence of stock prices as input and generating a corresponding market comment. Contrary to our expectations, the results show that prompts resembling programming languages yield better outcomes, whereas those similar to natural languages and longer formats, such as HTML and LaTeX, are less effective. Our findings offer insights into creating effective prompts for tasks that generate text from numerical sequences.
|
Masayuki Kawarada, Tatsuya Ishigaki, Hiroya Takamura |
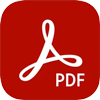
|
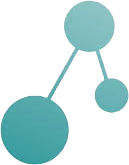 |
|
6/4/2024 |
RQ-RAG: Learning to Refine Queries for Retrieval Augmented Generation |
Large Language Models (LLMs) exhibit remarkable capabilities but are prone to generating inaccurate or hallucinatory responses. This limitation stems from their reliance on vast pretraining datasets, making them susceptible to errors in unseen scenarios. To tackle these challenges, Retrieval-Augmented Generation (RAG) addresses this by incorporating external, relevant documents into the response generation process, thus leveraging non-parametric knowledge alongside LLMs' in-context learning abilities. However, existing RAG implementations primarily focus on initial input for context retrieval, overlooking the nuances of ambiguous or complex queries that necessitate further clarification or decomposition for accurate responses. To this end, we propose learning to Refine Query for Retrieval Augmented Generation (RQ-RAG) in this paper, endeavoring to enhance the model by equipping it with capabilities for explicit rewriting, decomposition, and disambiguation. Our experimental results indicate that our method, when applied to a 7B Llama2 model, surpasses the previous state-of-the-art (SOTA) by an average of 1.9\% across three single-hop QA datasets, and also demonstrates enhanced performance in handling complex, multi-hop QA datasets. Our code is available at this https URL.
|
Chi-Min Chan, Chunpu Xu, Ruibin Yuan, Hongyin Luo, Wei Xue, Yike Guo, Jie Fu |
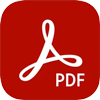
|
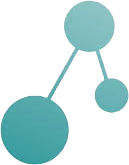 |
|
6/4/2024 |
Distillation for Multilingual Information Retrieval |
Recent work in cross-language information retrieval (CLIR), where queries and documents are in different languages, has shown the benefit of the Translate-Distill framework that trains a cross-language neural dual-encoder model using translation and distillation. However, Translate-Distill only supports a single document language. Multilingual information retrieval (MLIR), which ranks a multilingual document collection, is harder to train than CLIR because the model must assign comparable relevance scores to documents in different languages. This work extends Translate-Distill and propose Multilingual Translate-Distill (MTD) for MLIR. We show that ColBERT-X models trained with MTD outperform their counterparts trained ith Multilingual Translate-Train, which is the previous state-of-the-art training approach, by 5% to 25% in nDCG@20 and 15% to 45% in MAP. We also show that the model is robust to the way languages are mixed in training batches. Our implementation is available on GitHub.
|
Eugene Yang, Dawn Lawrie, James Mayfield |
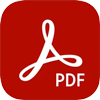
|
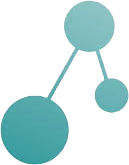 |
|
6/4/2024 |
PLAID SHIRTTT for Large-Scale Streaming Dense Retrieval |
PLAID, an efficient implementation of the ColBERT late interaction bi-encoder using pretrained language models for ranking, consistently achieves state-of-the-art performance in monolingual, cross-language, and multilingual retrieval. PLAID differs from ColBERT by assigning terms to clusters and representing those terms as cluster centroids plus compressed residual vectors. While PLAID is effective in batch experiments, its performance degrades in streaming settings where documents arrive over time because representations of new tokens may be poorly modeled by the earlier tokens used to select cluster centroids. PLAID Streaming Hierarchical Indexing that Runs on Terabytes of Temporal Text (PLAID SHIRTTT) addresses this concern using multi-phase incremental indexing based on hierarchical sharding. Experiments on ClueWeb09 and the multilingual NeuCLIR collection demonstrate the effectiveness of this approach both for the largest collection indexed to date by the ColBERT architecture and in the multilingual setting, respectively.
|
Dawn Lawrie, Efsun Kayi, Eugene Yang, James Mayfield, Douglas W. Oard |
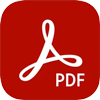
|
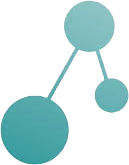 |
|
6/4/2024 |
Matryoshka Query Transformer for Large Vision-Language Models |
Large Vision-Language Models (LVLMs) typically encode an image into a fixed number of visual tokens (e.g., 576) and process these tokens with a language model. Despite their strong performance, LVLMs face challenges in adapting to varying computational constraints. This raises the question: can we achieve flexibility in the number of visual tokens to suit different tasks and computational resources? We answer this with an emphatic yes. Inspired by Matryoshka Representation Learning, we introduce the Matryoshka Query Transformer (MQT), capable of encoding an image into m visual tokens during inference, where m can be any number up to a predefined maximum. This is achieved by employing a query transformer with M latent query tokens to compress the visual embeddings. During each training step, we randomly select m <= M latent query tokens and train the model using only these first m tokens, discarding the rest. Combining MQT with LLaVA, we train a single model once, and flexibly and drastically reduce the number of inference-time visual tokens while maintaining similar or better performance compared to training independent models for each number of tokens. Our model, MQT-LLAVA, matches LLaVA-1.5 performance across 11 benchmarks using a maximum of 256 tokens instead of LLaVA's fixed 576. Reducing to 16 tokens (8x less TFLOPs) only sacrifices the performance by 2.4 points on MMBench. On certain tasks such as ScienceQA and MMMU, we can even go down to only 2 visual tokens with performance drops of just 3% and 6% each. Our exploration of the trade-off between the accuracy and computational cost brought about by the number of visual tokens facilitates future research to achieve the best of both worlds.
|
Wenbo Hu, Zi-Yi Dou, Liunian Harold Li, Amita Kamath, Nanyun Peng, Kai-Wei Chang |
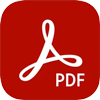
|
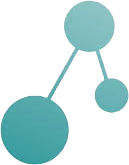 |
|
6/4/2024 |
Self-playing Adversarial Language Game Enhances LLM Reasoning |
We explore the self-play training procedure of large language models (LLMs) in a two-player adversarial language game called Adversarial Taboo. In this game, an attacker and a defender communicate with respect to a target word only visible to the attacker. The attacker aims to induce the defender to utter the target word unconsciously, while the defender tries to infer the target word from the attacker's utterances. To win the game, both players should have sufficient knowledge about the target word and high-level reasoning ability to infer and express in this information-reserved conversation. Hence, we are curious about whether LLMs' reasoning ability can be further enhanced by Self-Play in this Adversarial language Game (SPAG). With this goal, we let LLMs act as the attacker and play with a copy of itself as the defender on an extensive range of target words. Through reinforcement learning on the game outcomes, we observe that the LLMs' performance uniformly improves on a broad range of reasoning benchmarks. Furthermore, iteratively adopting this self-play process can continuously promote LLM's reasoning ability. The code is at this https URL.
|
Pengyu Cheng, Tianhao Hu, Han Xu, Zhisong Zhang, Yong Dai, Lei Han, Nan Du |
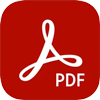
|
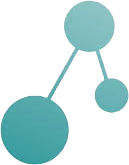 |
|
6/4/2024 |
Sparse Feature Circuits: Discovering and Editing Interpretable Causal Graphs in Language Models |
We introduce methods for discovering and applying sparse feature circuits. These are causally implicated subnetworks of human-interpretable features for explaining language model behaviors. Circuits identified in prior work consist of polysemantic and difficult-to-interpret units like attention heads or neurons, rendering them unsuitable for many downstream applications. In contrast, sparse feature circuits enable detailed understanding of unanticipated mechanisms. Because they are based on fine-grained units, sparse feature circuits are useful for downstream tasks: We introduce SHIFT, where we improve the generalization of a classifier by ablating features that a human judges to be task-irrelevant. Finally, we demonstrate an entirely unsupervised and scalable interpretability pipeline by discovering thousands of sparse feature circuits for automatically discovered model behaviors.
|
Samuel Marks, Can Rager, Eric J. Michaud, Yonatan Belinkov, David Bau, Aaron Mueller |
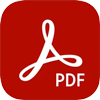
|
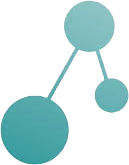 |
|
6/4/2024 |
Reporting Eye-Tracking Data Quality: Towards a New Standard |
Eye-tracking datasets are often shared in the format used by their creators for their original analyses, usually resulting in the exclusion of data considered irrelevant to the primary purpose. In order to increase re-usability of existing eye-tracking datasets for more diverse and initially not considered use cases, this work advocates a new approach of sharing eye-tracking data. Instead of publishing filtered and pre-processed datasets, the eye-tracking data at all pre-processing stages should be published together with data quality reports. In order to transparently report data quality and enable cross-dataset comparisons, we develop data quality reporting standards and metrics that can be automatically applied to a dataset, and integrate them into the open-source Python package pymovements (this https URL).
|
Deborah N. Jakobi, Daniel G. Krakowczyk, Lena A. Jäger |
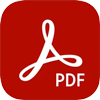
|
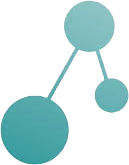 |
|
6/4/2024 |
Linear Attention Sequence Parallelism |
Sequence Parallel (SP) serves as a prevalent strategy to handle long sequences that exceed the memory limit of a single GPU. However, existing SP methods do not take advantage of linear attention features, resulting in sub-optimal parallelism efficiency and usability for linear attention-based language models. In this paper, we introduce Linear Attention Sequence Parallel (LASP), an efficient SP method tailored to linear attention-based language models. Specifically, we design an efficient point-to-point communication mechanism to leverage the right-product kernel trick of linear attention, which sharply decreases the communication overhead of SP. We also enhance the practical efficiency of LASP by performing kernel fusion and intermediate state caching, making the implementation of LASP hardware-friendly on GPU clusters. Furthermore, we meticulously ensure the compatibility of sequence-level LASP with all types of batch-level data parallel methods, which is vital for distributed training on large clusters with long sequences and large batches. We conduct extensive experiments on two linear attention-based models with varying sequence lengths and GPU cluster sizes. LASP scales sequence length up to 4096K using 128 A100 80G GPUs on 1B models, which is 8 times longer than existing SP methods while being significantly faster. The code is available at this https URL.
|
Weigao Sun, Zhen Qin, Dong Li, Xuyang Shen, Yu Qiao, Yiran Zhong |
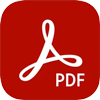
|
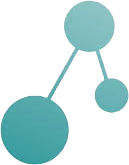 |
|
6/4/2024 |
Top Leaderboard Ranking = Top Coding Proficiency, Always? EvoEval: Evolving Coding Benchmarks via LLM |
LLMs have become the go-to choice for code generation tasks, with an exponential increase in the training, development, and usage of LLMs specifically for code generation. To evaluate the ability of LLMs on code, both academic and industry practitioners rely on popular handcrafted benchmarks. However, prior benchmarks contain only a very limited set of problems, both in quantity and variety. Further, due to popularity and age, many benchmarks are prone to data leakage where example solutions can be readily found on the web and thus potentially in training data. Such limitations inevitably lead us to inquire: Is the leaderboard performance on existing benchmarks reliable and comprehensive enough to measure the program synthesis ability of LLMs? To address this, we introduce EvoEval -- a program synthesis benchmark suite created by evolving existing benchmarks into different targeted domains for a comprehensive evaluation of LLM coding abilities. Our study on 51 LLMs shows that compared to the high performance obtained on standard benchmarks like HumanEval, there is a significant drop in performance (on average 39.4%) when using EvoEval. Additionally, the decrease in performance can range from 19.6% to 47.7%, leading to drastic ranking changes amongst LLMs and showing potential overfitting of existing benchmarks. Furthermore, we showcase various insights, including the brittleness of instruction-following models when encountering rewording or subtle changes as well as the importance of learning problem composition and decomposition. EvoEval not only provides comprehensive benchmarks, but can be used to further evolve arbitrary problems to keep up with advances and the ever-changing landscape of LLMs for code. We have open-sourced our benchmarks, tools, and complete LLM generations at this https URL
|
Chunqiu Steven Xia, Yinlin Deng, Lingming Zhang |
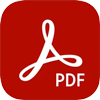
|
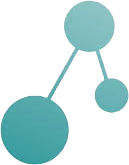 |
|
6/4/2024 |
Faithful Logical Reasoning via Symbolic Chain-of-Thought |
While the recent Chain-of-Thought (CoT) technique enhances the reasoning ability of large language models (LLMs) with the theory of mind, it might still struggle in handling logical reasoning that relies much on symbolic expressions and rigid deducing rules. To strengthen the logical reasoning capability of LLMs, we propose a novel Symbolic Chain-of-Thought, namely SymbCoT, a fully LLM-based framework that integrates symbolic expressions and logic rules with CoT prompting. Technically, building upon an LLM, SymbCoT 1) first translates the natural language context into the symbolic format, and then 2) derives a step-by-step plan to solve the problem with symbolic logical rules, 3) followed by a verifier to check the translation and reasoning chain. Via thorough evaluations on 5 standard datasets with both First-Order Logic and Constraint Optimization symbolic expressions, SymbCoT shows striking improvements over the CoT method consistently, meanwhile refreshing the current state-of-the-art performances. We further demonstrate that our system advances in more faithful, flexible, and explainable logical reasoning. To our knowledge, this is the first to combine symbolic expressions and rules into CoT for logical reasoning with LLMs. Code is open at this https URL.
|
Jundong Xu, Hao Fei, Liangming Pan, Qian Liu, Mong-Li Lee, Wynne Hsu |
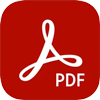
|
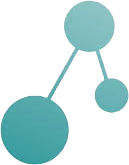 |
|
6/4/2024 |
CM-TTS: Enhancing Real Time Text-to-Speech Synthesis Efficiency through Weighted Samplers and Consistency Models |
Neural Text-to-Speech (TTS) systems find broad applications in voice assistants, e-learning, and audiobook creation. The pursuit of modern models, like Diffusion Models (DMs), holds promise for achieving high-fidelity, real-time speech synthesis. Yet, the efficiency of multi-step sampling in Diffusion Models presents challenges. Efforts have been made to integrate GANs with DMs, speeding up inference by approximating denoising distributions, but this introduces issues with model convergence due to adversarial training. To overcome this, we introduce CM-TTS, a novel architecture grounded in consistency models (CMs). Drawing inspiration from continuous-time diffusion models, CM-TTS achieves top-quality speech synthesis in fewer steps without adversarial training or pre-trained model dependencies. We further design weighted samplers to incorporate different sampling positions into model training with dynamic probabilities, ensuring unbiased learning throughout the entire training process. We present a real-time mel-spectrogram generation consistency model, validated through comprehensive evaluations. Experimental results underscore CM-TTS's superiority over existing single-step speech synthesis systems, representing a significant advancement in the field.
|
Xiang Li, Fan Bu, Ambuj Mehrish, Yingting Li, Jiale Han, Bo Cheng, Soujanya Poria |
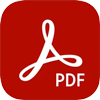
|
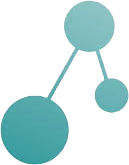 |
|
6/4/2024 |
Prepacking: A Simple Method for Fast Prefilling and Increased Throughput in Large Language Models |
During inference for transformer-based large language models (LLM), prefilling is the computation of the key-value (KV) cache for input tokens in the prompt prior to autoregressive generation. For longer input prompt lengths, prefilling will incur a significant overhead on decoding time. In this work, we highlight the following pitfall of prefilling: for batches containing high-varying prompt lengths, significant computation is wasted by the standard practice of padding sequences to the maximum length. As LLMs increasingly support longer context lengths, potentially up to 10 million tokens, variations in prompt lengths within a batch become more pronounced. To address this, we propose Prepacking, a simple yet effective method to optimize prefilling computation. To avoid redundant computation on pad tokens, prepacking combines prompts of varying lengths into a sequence and packs multiple sequences into a compact batch using a bin-packing algorithm. It then modifies the attention mask and positional encoding to compute multiple prefilled KV-caches for multiple prompts within a single sequence. On standard curated dataset containing prompts with varying lengths, we obtain a significant speed and memory efficiency improvements as compared to the default padding-based prefilling computation within Huggingface across a range of base model configurations and inference serving scenarios.
|
Siyan Zhao, Daniel Israel, Guy Van den Broeck, Aditya Grover |
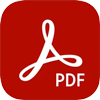
|
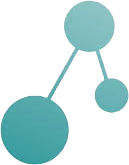 |
|
6/4/2024 |
Conifer: Improving Complex Constrained Instruction-Following Ability of Large Language Models |
The ability of large language models (LLMs) to follow instructions is crucial to real-world applications. Despite recent advances, several studies have highlighted that LLMs struggle when faced with challenging instructions, especially those that include complex constraints, hindering their effectiveness in various tasks. To address this challenge, we introduce Conifer, a novel instruction tuning dataset, designed to enhance LLMs to follow multi-level instructions with complex constraints. Utilizing GPT-4, we curate the dataset by a series of LLM-driven refinement processes to ensure high quality. We also propose a progressive learning scheme that emphasizes an easy-to-hard progression, and learning from process feedback. Models trained with Conifer exhibit remarkable improvements in instruction-following abilities, especially for instructions with complex constraints. On several instruction-following benchmarks, our 7B model outperforms the state-of-the-art open-source 7B models, even exceeds the performance of models 10 times larger on certain metrics. All the code and Conifer dataset are available at this https URL.
|
Haoran Sun, Lixin Liu, Junjie Li, Fengyu Wang, Baohua Dong, Ran Lin, Ruohui Huang |
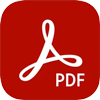
|
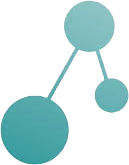 |
|
6/4/2024 |
PLAYER*: Enhancing LLM-based Multi-Agent Communication and Interaction in Murder Mystery Games |
Recent advancements in Large Language Models (LLMs) have enhanced the efficacy of agent communication and social interactions. Despite these advancements, building LLM-based agents for reasoning in dynamic environments involving competition and collaboration remains challenging due to the limitations of informed graph-based search methods. We propose PLAYER*, a novel framework based on an anytime sampling-based planner, which utilises sensors and pruners to enable a purely question-driven searching framework for complex reasoning tasks. We also introduce a quantifiable evaluation method using multiple-choice questions and construct the WellPlay dataset with 1,482 QA pairs. Experiments demonstrate PLAYER*'s efficiency and performance enhancements compared to existing methods in complex, dynamic environments with quantifiable results.
|
Qinglin Zhu, Runcong Zhao, Jinhua Du, Lin Gui, Yulan He |
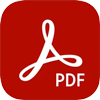
|
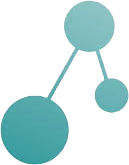 |
|
6/4/2024 |
From Matching to Generation: A Survey on Generative Information Retrieval |
Information Retrieval (IR) systems are crucial tools for users to access information, widely applied in scenarios like search engines, question answering, and recommendation systems. Traditional IR methods, based on similarity matching to return ranked lists of documents, have been reliable means of information acquisition, dominating the IR field for years. With the advancement of pre-trained language models, generative information retrieval (GenIR) has emerged as a novel paradigm, gaining increasing attention in recent years. Currently, research in GenIR can be categorized into two aspects: generative document retrieval (GR) and reliable response generation. GR leverages the generative model's parameters for memorizing documents, enabling retrieval by directly generating relevant document identifiers without explicit indexing. Reliable response generation, on the other hand, employs language models to directly generate the information users seek, breaking the limitations of traditional IR in terms of document granularity and relevance matching, offering more flexibility, efficiency, and creativity, thus better meeting practical needs. This paper aims to systematically review the latest research progress in GenIR. We will summarize the advancements in GR regarding model training, document identifier, incremental learning, downstream tasks adaptation, multi-modal GR and generative recommendation, as well as progress in reliable response generation in aspects of internal knowledge memorization, external knowledge augmentation, generating response with citations and personal information assistant. We also review the evaluation, challenges and future prospects in GenIR systems. This review aims to offer a comprehensive reference for researchers in the GenIR field, encouraging further development in this area.
|
Xiaoxi Li, Jiajie Jin, Yujia Zhou, Yuyao Zhang, Peitian Zhang, Yutao Zhu, Zhicheng Dou |
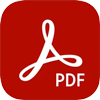
|
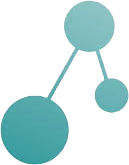 |
|
6/4/2024 |
Unsolvable Problem Detection: Evaluating Trustworthiness of Vision Language Models |
This paper introduces a novel and significant challenge for Vision Language Models (VLMs), termed Unsolvable Problem Detection (UPD). UPD examines the VLM's ability to withhold answers when faced with unsolvable problems in the context of Visual Question Answering (VQA) tasks. UPD encompasses three distinct settings: Absent Answer Detection (AAD), Incompatible Answer Set Detection (IASD), and Incompatible Visual Question Detection (IVQD). To deeply investigate the UPD problem, extensive experiments indicate that most VLMs, including GPT-4V and LLaVA-Next-34B, struggle with our benchmarks to varying extents, highlighting significant room for the improvements. To address UPD, we explore both training-free and training-based solutions, offering new insights into their effectiveness and limitations. We hope our insights, together with future efforts within the proposed UPD settings, will enhance the broader understanding and development of more practical and reliable VLMs.
|
Atsuyuki Miyai, Jingkang Yang, Jingyang Zhang, Yifei Ming, Qing Yu, Go Irie, Yixuan Li, Hai Li, Ziwei Liu, Kiyoharu Aizawa |
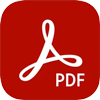
|
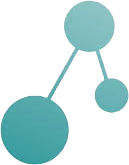 |
|
6/4/2024 |
KnowHalu: Hallucination Detection via Multi-Form Knowledge Based Factual Checking |
This paper introduces KnowHalu, a novel approach for detecting hallucinations in text generated by large language models (LLMs), utilizing step-wise reasoning, multi-formulation query, multi-form knowledge for factual checking, and fusion-based detection mechanism. As LLMs are increasingly applied across various domains, ensuring that their outputs are not hallucinated is critical. Recognizing the limitations of existing approaches that either rely on the self-consistency check of LLMs or perform post-hoc fact-checking without considering the complexity of queries or the form of knowledge, KnowHalu proposes a two-phase process for hallucination detection. In the first phase, it identifies non-fabrication hallucinations--responses that, while factually correct, are irrelevant or non-specific to the query. The second phase, multi-form based factual checking, contains five key steps: reasoning and query decomposition, knowledge retrieval, knowledge optimization, judgment generation, and judgment aggregation. Our extensive evaluations demonstrate that KnowHalu significantly outperforms SOTA baselines in detecting hallucinations across diverse tasks, e.g., improving by 15.65% in QA tasks and 5.50% in summarization tasks, highlighting its effectiveness and versatility in detecting hallucinations in LLM-generated content.
|
Jiawei Zhang, Chejian Xu, Yu Gai, Freddy Lecue, Dawn Song, Bo Li |
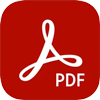
|
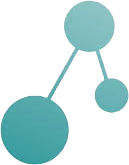 |
|
6/4/2024 |
MineLand: Simulating Large-Scale Multi-Agent Interactions with Limited Multimodal Senses and Physical Needs |
Conventional multi-agent simulators often assume perfect information and limitless capabilities, hindering the ecological validity of social interactions. We propose a multi-agent Minecraft simulator, MineLand, that bridges this gap by introducing limited multimodal senses and physical needs. Our simulator supports up to 48 agents with limited visual, auditory, and environmental awareness, forcing them to actively communicate and collaborate to fulfill physical needs like food and resources. This fosters dynamic and valid multi-agent interactions. We further introduce an AI agent framework, Alex, inspired by multitasking theory, enabling agents to handle intricate coordination and scheduling. Our experiments demonstrate that the simulator, the corresponding benchmark, and the AI agent framework contribute to more ecological and nuanced collective behavior. The source code of MineLand and Alex is openly available at this https URL.
|
Xianhao Yu, Jiaqi Fu, Renjia Deng, Wenjuan Han |
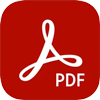
|
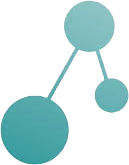 |
|
6/4/2024 |
Can Language Beat Numerical Regression? Language-Based Multimodal Trajectory Prediction |
Language models have demonstrated impressive ability in context understanding and generative performance. Inspired by the recent success of language foundation models, in this paper, we propose LMTraj (Language-based Multimodal Trajectory predictor), which recasts the trajectory prediction task into a sort of question-answering problem. Departing from traditional numerical regression models, which treat the trajectory coordinate sequence as continuous signals, we consider them as discrete signals like text prompts. Specially, we first transform an input space for the trajectory coordinate into the natural language space. Here, the entire time-series trajectories of pedestrians are converted into a text prompt, and scene images are described as text information through image captioning. The transformed numerical and image data are then wrapped into the question-answering template for use in a language model. Next, to guide the language model in understanding and reasoning high-level knowledge, such as scene context and social relationships between pedestrians, we introduce an auxiliary multi-task question and answering. We then train a numerical tokenizer with the prompt data. We encourage the tokenizer to separate the integer and decimal parts well, and leverage it to capture correlations between the consecutive numbers in the language model. Lastly, we train the language model using the numerical tokenizer and all of the question-answer prompts. Here, we propose a beam-search-based most-likely prediction and a temperature-based multimodal prediction to implement both deterministic and stochastic inferences. Applying our LMTraj, we show that the language-based model can be a powerful pedestrian trajectory predictor, and outperforms existing numerical-based predictor methods. Code is publicly available at this https URL .
|
Inhwan Bae, Junoh Lee, Hae-Gon Jeon |
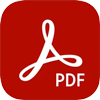
|
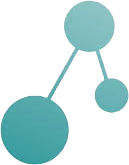 |
|
6/4/2024 |
SpaceByte: Towards Deleting Tokenization from Large Language Modeling |
Tokenization is widely used in large language models because it significantly improves performance. However, tokenization imposes several disadvantages, such as performance biases, increased adversarial vulnerability, decreased character-level modeling performance, and increased modeling complexity. To address these disadvantages without sacrificing performance, we propose SpaceByte, a novel byte-level decoder architecture that closes the performance gap between byte-level and subword autoregressive language modeling. SpaceByte consists of a byte-level Transformer model, but with extra larger transformer blocks inserted in the middle of the layers. We find that performance is significantly improved by applying these larger blocks only after certain bytes, such as space characters, which typically denote word boundaries. Our experiments show that for a fixed training and inference compute budget, SpaceByte outperforms other byte-level architectures and roughly matches the performance of tokenized Transformer architectures.
|
Kevin Slagle |
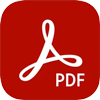
|
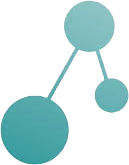 |
|
6/4/2024 |
Introducing v0.5 of the AI Safety Benchmark from MLCommons |
This paper introduces v0.5 of the AI Safety Benchmark, which has been created by the MLCommons AI Safety Working Group. The AI Safety Benchmark has been designed to assess the safety risks of AI systems that use chat-tuned language models. We introduce a principled approach to specifying and constructing the benchmark, which for v0.5 covers only a single use case (an adult chatting to a general-purpose assistant in English), and a limited set of personas (i.e., typical users, malicious users, and vulnerable users). We created a new taxonomy of 13 hazard categories, of which 7 have tests in the v0.5 benchmark. We plan to release version 1.0 of the AI Safety Benchmark by the end of 2024. The v1.0 benchmark will provide meaningful insights into the safety of AI systems. However, the v0.5 benchmark should not be used to assess the safety of AI systems. We have sought to fully document the limitations, flaws, and challenges of v0.5. This release of v0.5 of the AI Safety Benchmark includes (1) a principled approach to specifying and constructing the benchmark, which comprises use cases, types of systems under test (SUTs), language and context, personas, tests, and test items; (2) a taxonomy of 13 hazard categories with definitions and subcategories; (3) tests for seven of the hazard categories, each comprising a unique set of test items, i.e., prompts. There are 43,090 test items in total, which we created with templates; (4) a grading system for AI systems against the benchmark; (5) an openly available platform, and downloadable tool, called ModelBench that can be used to evaluate the safety of AI systems on the benchmark; (6) an example evaluation report which benchmarks the performance of over a dozen openly available chat-tuned language models; (7) a test specification for the benchmark.
|
Bertie Vidgen, Adarsh Agrawal, Ahmed M. Ahmed, Victor Akinwande, Namir Al-Nuaimi, Najla Alfaraj, Elie Alhajjar, Lora Aroyo, Trupti Bavalatti, Borhane Blili-Hamelin, Kurt Bollacker, Rishi Bomassani, Marisa Ferrara Boston, Siméon Campos, Kal Chakra, Canyu Chen, Cody Coleman, Zacharie Delpierre Coudert, Leon Derczynski, Debojyoti Dutta, Ian Eisenberg, James Ezick, Heather Frase, Brian Fuller, Ram Gandikota, Agasthya Gangavarapu, Ananya Gangavarapu, James Gealy, Rajat Ghosh, James Goel, Usman Gohar, Sujata Goswami, Scott A. Hale, Wiebke Hutiri, Joseph Marvin Imperial, Surgan Jandial, Nick Judd, Felix Juefei-Xu, Foutse Khomh, Bhavya Kailkhura, Hannah Rose Kirk, Kevin Klyman, Chris Knotz, Michael Kuchnik, Shachi H. Kumar, Chris Lengerich, Bo Li, Zeyi Liao, Eileen Peters Long, Victor Lu, Yifan Mai, Priyanka Mary Mammen, Kelvin Manyeki, Sean McGregor, Virendra Mehta, Shafee Mohammed, Emanuel Moss, Lama Nachman, Dinesh Jinenhally Naganna, Amin Nikanjam, Besmira Nushi, Luis Oala, Iftach Orr, Alicia Parrish, Cigdem Patlak, William Pietri, Forough Poursabzi-Sangdeh, Eleonora Presani, Fabrizio Puletti, Paul Röttger, Saurav Sahay, Tim Santos, Nino Scherrer, Alice Schoenauer Sebag, Patrick Schramowski, Abolfazl Shahbazi, Vin Sharma, Xudong Shen, Vamsi Sistla, Leonard Tang, Davide Testuggine, Vithursan Thangarasa, Elizabeth Anne Watkins, Rebecca Weiss, Chris Welty, Tyler Wilbers, Adina Williams, Carole-Jean Wu, Poonam Yadav, Xianjun Yang, Yi Zeng, Wenhui Zhang, Fedor Zhdanov, Jiacheng Zhu, Percy Liang, Peter Mattson, Joaquin Vanschoren |
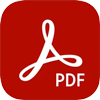
|
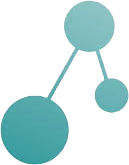 |
|
6/4/2024 |
Spatio-Temporal Side Tuning Pre-trained Foundation Models for Video-based Pedestrian Attribute Recognition |
Existing pedestrian attribute recognition (PAR) algorithms are mainly developed based on a static image, however, the performance is unreliable in challenging scenarios, such as heavy occlusion, motion blur, etc. In this work, we propose to understand human attributes using video frames that can fully use temporal information by fine-tuning a pre-trained multi-modal foundation model efficiently. Specifically, we formulate the video-based PAR as a vision-language fusion problem and adopt a pre-trained foundation model CLIP to extract the visual features. More importantly, we propose a novel spatiotemporal side-tuning strategy to achieve parameter-efficient optimization of the pre-trained vision foundation model. To better utilize the semantic information, we take the full attribute list that needs to be recognized as another input and transform the attribute words/phrases into the corresponding sentence via split, expand, and prompt operations. Then, the text encoder of CLIP is utilized for embedding processed attribute descriptions. The averaged visual tokens and text tokens are concatenated and fed into a fusion Transformer for multi-modal interactive learning. The enhanced tokens will be fed into a classification head for pedestrian attribute prediction. Extensive experiments on two large-scale video-based PAR datasets fully validated the effectiveness of our proposed framework. The source code of this paper is available at this https URL.
|
Xiao Wang, Qian Zhu, Jiandong Jin, Jun Zhu, Futian Wang, Bo Jiang, Yaowei Wang, Yonghong Tian |
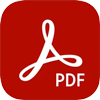
|
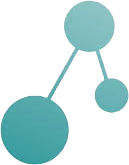 |
|
6/4/2024 |
Weak-to-Strong Extrapolation Expedites Alignment |
Although the capabilities of large language models (LLMs) ideally scale up with increasing data and compute, they are inevitably constrained by limited resources in reality. Suppose we have a moderately trained LLM (e.g., trained to align with human preference) in hand, can we further exploit its potential and cheaply acquire a stronger model? In this paper, we propose a simple method called ExPO to boost LLMs' alignment with human preference. ExPO assumes that a medium-aligned model can be interpolated between a less-aligned (weaker) model, e.g., the initial SFT model, and a better-aligned (stronger) one, thereby directly obtaining this stronger model by extrapolating from the weights of the former two relatively weaker models. On the AlpacaEval 2.0 benchmark, we show that ExPO pushes models trained with less preference data (e.g., 10% or 20%) to reach and even surpass the fully-trained one, without any additional training. Furthermore, ExPO also significantly improves off-the-shelf DPO/RLHF models and exhibits decent scalability across model sizes from 7B to 70B. Our work demonstrates the efficacy of model extrapolation in exploiting LLMs' capabilities, suggesting a promising direction that deserves future exploration.
|
Chujie Zheng, Ziqi Wang, Heng Ji, Minlie Huang, Nanyun Peng |
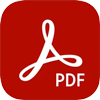
|
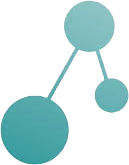 |
|
6/4/2024 |
Causal Evaluation of Language Models |
Causal reasoning is viewed as crucial for achieving human-level machine intelligence. Recent advances in language models have expanded the horizons of artificial intelligence across various domains, sparking inquiries into their potential for causal reasoning. In this work, we introduce Causal evaluation of Language Models (CaLM), which, to the best of our knowledge, is the first comprehensive benchmark for evaluating the causal reasoning capabilities of language models. First, we propose the CaLM framework, which establishes a foundational taxonomy consisting of four modules: causal target (i.e., what to evaluate), adaptation (i.e., how to obtain the results), metric (i.e., how to measure the results), and error (i.e., how to analyze the bad results). This taxonomy defines a broad evaluation design space while systematically selecting criteria and priorities. Second, we compose the CaLM dataset, comprising 126,334 data samples, to provide curated sets of causal targets, adaptations, metrics, and errors, offering extensive coverage for diverse research pursuits. Third, we conduct an extensive evaluation of 28 leading language models on a core set of 92 causal targets, 9 adaptations, 7 metrics, and 12 error types. Fourth, we perform detailed analyses of the evaluation results across various dimensions (e.g., adaptation, scale). Fifth, we present 50 high-level empirical findings across 9 dimensions (e.g., model), providing valuable guidance for future language model development. Finally, we develop a multifaceted platform, including a website, leaderboards, datasets, and toolkits, to support scalable and adaptable assessments. We envision CaLM as an ever-evolving benchmark for the community, systematically updated with new causal targets, adaptations, models, metrics, and error types to reflect ongoing research advancements. Project website is at this https URL.
|
Sirui Chen, Bo Peng, Meiqi Chen, Ruiqi Wang, Mengying Xu, Xingyu Zeng, Rui Zhao, Shengjie Zhao, Yu Qiao, Chaochao Lu |
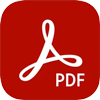
|
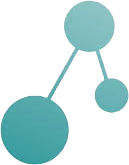 |
|
6/4/2024 |
How Much are LLMs Contaminated? A Comprehensive Survey and the LLMSanitize Library |
With the rise of Large Language Models (LLMs) in recent years, new opportunities are emerging, but also new challenges, and contamination is quickly becoming critical. Business applications and fundraising in AI have reached a scale at which a few percentage points gained on popular question-answering benchmarks could translate into dozens of millions of dollars, placing high pressure on model integrity. At the same time, it is becoming harder and harder to keep track of the data that LLMs have seen; if not impossible with closed-source models like GPT-4 and Claude-3 not divulging any information on the training set. As a result, contamination becomes a critical issue: LLMs' performance may not be reliable anymore, as the high performance may be at least partly due to their previous exposure to the data. This limitation jeopardizes the entire progress in the field of NLP, yet, there remains a lack of methods on how to efficiently address contamination, or a clear consensus on prevention, mitigation and classification of contamination. In this paper, we survey all recent work on contamination with LLMs, and help the community track contamination levels of LLMs by releasing an open-source Python library named LLMSanitize implementing major contamination detection algorithms, which link is: this https URL.
|
Mathieu Ravaut, Bosheng Ding, Fangkai Jiao, Hailin Chen, Xingxuan Li, Ruochen Zhao, Chengwei Qin, Caiming Xiong, Shafiq Joty |
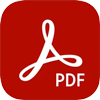
|
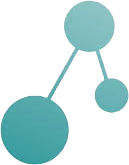 |
|
6/4/2024 |
Let's Think Dot by Dot: Hidden Computation in Transformer Language Models |
Chain-of-thought responses from language models improve performance across most benchmarks. However, it remains unclear to what extent these performance gains can be attributed to human-like task decomposition or simply the greater computation that additional tokens allow. We show that transformers can use meaningless filler tokens (e.g., '......') in place of a chain of thought to solve two hard algorithmic tasks they could not solve when responding without intermediate tokens. However, we find empirically that learning to use filler tokens is difficult and requires specific, dense supervision to converge. We also provide a theoretical characterization of the class of problems where filler tokens are useful in terms of the quantifier depth of a first-order formula. For problems satisfying this characterization, chain-of-thought tokens need not provide information about the intermediate computational steps involved in multi-token computations. In summary, our results show that additional tokens can provide computational benefits independent of token choice. The fact that intermediate tokens can act as filler tokens raises concerns about large language models engaging in unauditable, hidden computations that are increasingly detached from the observed chain-of-thought tokens.
|
Jacob Pfau, William Merrill, Samuel R. Bowman |
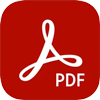
|
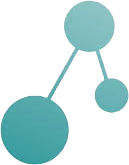 |
|
6/4/2024 |
FABLES: Evaluating faithfulness and content selection in book-length summarization |
While long-context large language models (LLMs) can technically summarize book-length documents (>100K tokens), the length and complexity of the documents have so far prohibited evaluations of input-dependent aspects like faithfulness. In this paper, we conduct the first large-scale human evaluation of faithfulness and content selection on LLM-generated summaries of fictional books. Our study mitigates the issue of data contamination by focusing on summaries of books published in 2023 or 2024, and we hire annotators who have fully read each book prior to the annotation task to minimize cost and cognitive burden. We collect FABLES, a dataset of annotations on 3,158 claims made in LLM-generated summaries of 26 books, at a cost of $5.2K USD, which allows us to rank LLM summarizers based on faithfulness: Claude-3-Opus significantly outperforms all closed-source LLMs, while the open-source Mixtral is on par with GPT-3.5-Turbo. An analysis of the annotations reveals that most unfaithful claims relate to events and character states, and they generally require indirect reasoning over the narrative to invalidate. While LLM-based auto-raters have proven reliable for factuality and coherence in other settings, we implement several LLM raters of faithfulness and find that none correlates strongly with human annotations, especially with regard to detecting unfaithful claims. Our experiments suggest that detecting unfaithful claims is an important future direction not only for summarization evaluation but also as a testbed for long-context understanding. Finally, we move beyond faithfulness by exploring content selection errors in book-length summarization: we develop a typology of omission errors related to crucial narrative elements and also identify a systematic over-emphasis on events occurring towards the end of the book.
|
Yekyung Kim, Yapei Chang, Marzena Karpinska, Aparna Garimella, Varun Manjunatha, Kyle Lo, Tanya Goyal, Mohit Iyyer |
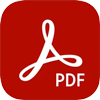
|
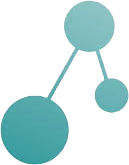 |
|
6/4/2024 |
Grokked Transformers are Implicit Reasoners: A Mechanistic Journey to the Edge of Generalization |
We study whether transformers can learn to implicitly reason over parametric knowledge, a skill that even the most capable language models struggle with. Focusing on two representative reasoning types, composition and comparison, we consistently find that transformers can learn implicit reasoning, but only through grokking, i.e., extended training far beyond overfitting. The levels of generalization also vary across reasoning types: when faced with out-of-distribution examples, transformers fail to systematically generalize for composition but succeed for comparison. We delve into the model's internals throughout training, conducting analytical experiments that reveal: 1) the mechanism behind grokking, such as the formation of the generalizing circuit and its relation to the relative efficiency of generalizing and memorizing circuits, and 2) the connection between systematicity and the configuration of the generalizing circuit. Our findings guide data and training setup to better induce implicit reasoning and suggest potential improvements to the transformer architecture, such as encouraging cross-layer knowledge sharing. Furthermore, we demonstrate that for a challenging reasoning task with a large search space, GPT-4-Turbo and Gemini-1.5-Pro based on non-parametric memory fail badly regardless of prompting styles or retrieval augmentation, while a fully grokked transformer can achieve near-perfect accuracy, showcasing the power of parametric memory for complex reasoning.
|
Boshi Wang, Xiang Yue, Yu Su, Huan Sun |
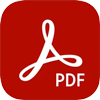
|
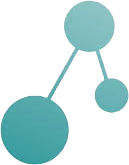 |
|
6/4/2024 |
Tool Learning with Large Language Models: A Survey |
Recently, tool learning with large language models (LLMs) has emerged as a promising paradigm for augmenting the capabilities of LLMs to tackle highly complex problems. Despite growing attention and rapid advancements in this field, the existing literature remains fragmented and lacks systematic organization, posing barriers to entry for newcomers. This gap motivates us to conduct a comprehensive survey of existing works on tool learning with LLMs. In this survey, we focus on reviewing existing literature from the two primary aspects (1) why tool learning is beneficial and (2) how tool learning is implemented, enabling a comprehensive understanding of tool learning with LLMs. We first explore the "why" by reviewing both the benefits of tool integration and the inherent benefits of the tool learning paradigm from six specific aspects. In terms of "how", we systematically review the literature according to a taxonomy of four key stages in the tool learning workflow: task planning, tool selection, tool calling, and response generation. Additionally, we provide a detailed summary of existing benchmarks and evaluation methods, categorizing them according to their relevance to different stages. Finally, we discuss current challenges and outline potential future directions, aiming to inspire both researchers and industrial developers to further explore this emerging and promising area. We also maintain a GitHub repository to continually keep track of the relevant papers and resources in this rising area at \url{this https URL}.
|
Changle Qu, Sunhao Dai, Xiaochi Wei, Hengyi Cai, Shuaiqiang Wang, Dawei Yin, Jun Xu, Ji-Rong Wen |
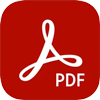
|
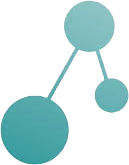 |
|
6/4/2024 |
Self-Explore to Avoid the Pit: Improving the Reasoning Capabilities of Language Models with Fine-grained Rewards |
Training on large amounts of rationales (i.e., CoT Fine-tuning) is effective at improving the reasoning capabilities of large language models (LLMs). However, acquiring human-authored rationales or augmenting rationales from proprietary models is costly and not scalable. In this paper, we study the problem of whether LLMs could self-improve their reasoning capabilities. To this end, we propose Self-Explore, where the LLM is tasked to explore the first wrong step (i.e., the first pit) within the rationale and use such signals as fine-grained rewards for further improvement. On the GSM8K and MATH test set, Self-Explore achieves 11.57% and 2.89% improvement on average across three LLMs compared to supervised fine-tuning (SFT). Our code is available at this https URL.
|
Hyeonbin Hwang, Doyoung Kim, Seungone Kim, Seonghyeon Ye, Minjoon Seo |
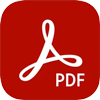
|
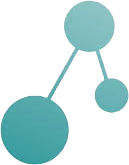 |
|
6/4/2024 |
Token-level Direct Preference Optimization |
Fine-tuning pre-trained Large Language Models (LLMs) is essential to align them with human values and intentions. This process often utilizes methods like pairwise comparisons and KL divergence against a reference LLM, focusing on the evaluation of full answers generated by the models. However, the generation of these responses occurs in a token level, following a sequential, auto-regressive fashion. In this paper, we introduce Token-level Direct Preference Optimization (TDPO), a novel approach to align LLMs with human preferences by optimizing policy at the token level. Unlike previous methods, which face challenges in divergence efficiency, TDPO incorporates forward KL divergence constraints for each token, improving alignment and diversity. Utilizing the Bradley-Terry model for a token-based reward system, TDPO enhances the regulation of KL divergence, while preserving simplicity without the need for explicit reward modeling. Experimental results across various text tasks demonstrate TDPO's superior performance in balancing alignment with generation diversity. Notably, fine-tuning with TDPO strikes a better balance than DPO in the controlled sentiment generation and single-turn dialogue datasets, and significantly improves the quality of generated responses compared to both DPO and PPO-based RLHF methods. Our code is open-sourced at this https URL.
|
Yongcheng Zeng, Guoqing Liu, Weiyu Ma, Ning Yang, Haifeng Zhang, Jun Wang |
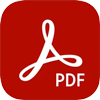
|
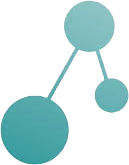 |
|
6/4/2024 |
The VoicePrivacy 2024 Challenge Evaluation Plan |
The task of the challenge is to develop a voice anonymization system for speech data which conceals the speaker's voice identity while protecting linguistic content and emotional states. The organizers provide development and evaluation datasets and evaluation scripts, as well as baseline anonymization systems and a list of training resources formed on the basis of the participants' requests. Participants apply their developed anonymization systems, run evaluation scripts and submit evaluation results and anonymized speech data to the organizers. Results will be presented at a workshop held in conjunction with Interspeech 2024 to which all participants are invited to present their challenge systems and to submit additional workshop papers.
|
Natalia Tomashenko, Xiaoxiao Miao, Pierre Champion, Sarina Meyer, Xin Wang, Emmanuel Vincent, Michele Panariello, Nicholas Evans, Junichi Yamagishi, Massimiliano Todisco |
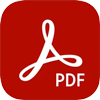
|
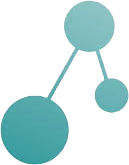 |
|